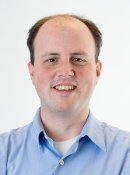
This month’s AI magazine includes the article “DARPA’s Explainable Artificial Intelligence Program,” which mentions Michigan Tech’s DARPA research. ICC member Shane Mueller is principal investigator of a 4-year, $255K DARPA XAI project.
The section of the article “Naturalistic Decision-Making Foundations of XAI” reads: “The objective of the IHMC team (which includes researchers from MacroCognition and Michigan Technological University) is to develop and evaluate psychologically plausible models of explanation and develop actionable concepts, methods, measures, and metrics for explanatory reasoning. The IHMC team is investigating the nature of explanation itself.”
Abstract: Dramatic success in machine learning has led to a new wave of AI applications (for example, transportation, security, medicine, finance, defense) that offer tremendous benefits but cannot explain their decisions and actions to human users. DARPA’s explainable artificial intelligence (XAI) program endeavors to create AI systems whose learned models and decisions can be understood and appropriately trusted by end users. Realizing this goal requires methods for learning more explainable models, designing effective explanation interfaces, and understanding the psychologic requirements for effective explanations. The XAI developer teams are addressing the first two challenges by creating ML techniques and developing principles, strategies, and human-computer interaction techniques for generating effective explanations. Another XAI team is addressing the third challenge by summarizing, extending, and applying psychologic theories of explanation to help the XAI evaluator define a suitable evaluation framework, which the developer teams will use to test their systems. The XAI teams completed the first of this 4-year program in May 2018. In a series of ongoing evaluations, the developer teams are assessing how well their XAM systems’ explanations improve user understanding, user trust, and user task performance.
https://www.aaai.org/ojs/index.php/aimagazine/article/view/2850
https://doi.org/10.1609/aimag.v40i2.2850