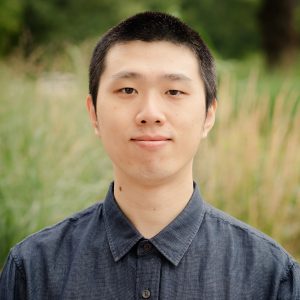
Dr. Xiaoyong (Brian) Yuan, assistant professor in both the Computer Science and Applied Computing departments at Michigan Tech, will present a lecture on Friday, February 18, 2022, at 3:00 pm, in Rekhi Hall Room 214. The lecture can also be attended virtually via Zoom.
The title of Dr. Yaun’s lecture is, “Towards Trustworthy Machine Learning: Security, Privacy, and Safety.”
Following Yuan’s talk, Dr. Susanta Ghosh (Mechanical Engineering-Engineering Mechanics) will also present a lecture. His research interests include scientific machine learning; computational mechanics; uncertainty quantification; inverse problems; and high-performance computing. Ghosh directs the Computational Mechanics and Machine Learning Lab. See his talk abstract and bio below.
Dr. Yuan’s research interests span the fields of deep learning, machine learning, security and privacy, and cloud computing. He has published papers in top-tier journals and conference proceedings, such as IEEE Transactions on Neural Networks and Learning Systems (TNNLS) and the AAAI Conference on Artificial Intelligence. And he has served as a reviewer for several leading journals and conferences, such as IEEE Transactions on Neural Networks and Learning Systems (TNNLS), International Conference on Learning Representations (ICLR), IEEE Transactions on Dependable and Secure Computing (TDSC), and IEEE Transactions on Parallel and Distributed Systems (TPDS).
Link to Dr. Yuan’s website here.
Lecture Title and Abstract: Susanta Ghosh
Abstract: In this talk, I will present our recent progress on the development of machine learning models for medical-image classification problems. Despite the promise of Convolutional neural network (CNN) based classification models for histopathological images, it is infeasible to quantify its uncertainties. Moreover, CNNs may suffer from overfitting when the data is biased. We show that Bayesian–CNN can overcome these limitations by regularizing automatically and by quantifying the uncertainty. We have developed a novel technique to utilize the uncertainties provided by the Bayesian–CNN that significantly improves the performance on a large fraction of the test data (about 6% improvement in accuracy on 77% of test data). Further, we provide a novel explanation for the uncertainty quantified by the Bayesian—CNNs via projecting the data into a low dimensional space through a nonlinear dimensionality reduction technique.
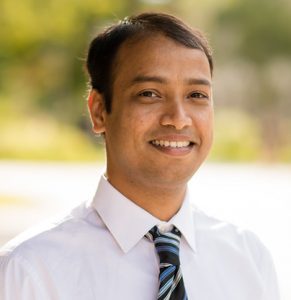
Bio: Susanta Ghosh is an assistant professor in the Mechanical Engineering-Engineering Mechanics at the Michigan Technological University. He is also a Faculty member of Center for Data Sciences at Michigan Tech. Prior to joining Michigan Tech he has worked as an Associate in Research at the Duke University. He was a postdoctoral scholar at the University of Michigan. Prior to that he was a research fellow at the Technical University of Catalunya, Barcelona, Spain. His M.S. and Ph.D. degrees are from the Indian Institute of Science, Bangalore, and BS degree from Indian Institute of Engineering Science and Technology, Shibpur. His research interest lies in the areas of Scientific Machine Learning and Uncertainty Quantification applied to materials modeling and medical imaging. He is also active in Computational Mechanics.
The lecture is sponsored by the Michigan Tech Department of Computer Science.