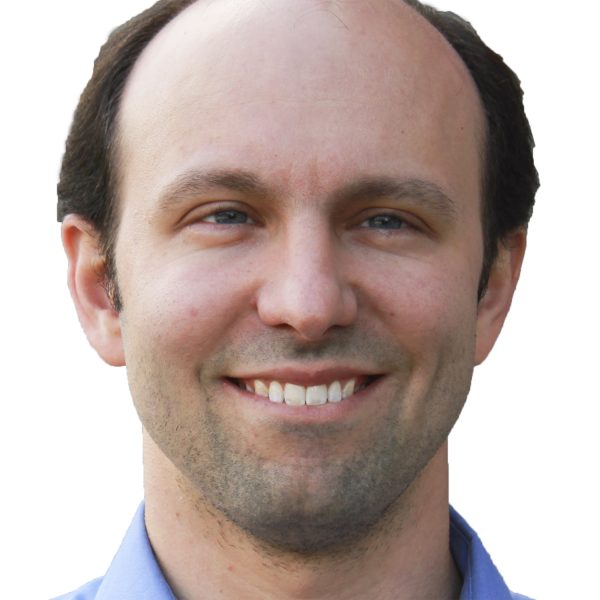
Daniel Neill of New York University will present a Computer Science Colloquium lecture on December 2, 2022, at 3:00 p.m., via Zoom. The talk is presented by the Department of Computer Science.
The title of Neill’s talk is, “Machine Learning and Event Detection for Urban Public Health.”
Abstract: This talk will present new machine learning algorithms which can assist cities in monitoring and improving population health. First, we describe new approaches for targeting health interventions to address the opioid crisis. Our methods provide situational awareness of emerging patterns of overdoses, opioid use disorder, and risk behaviors at the geographic, subpopulation, and individual levels, as well as identifying networks of physicians and dispensaries engaged in unsafe opioid prescribing behaviors.
Second, we describe a new event detection approach that provides public health practitioners with a “safety net” to detect newly emerging disease outbreaks and other previously unknown but potentially relevant patterns, using free-text chief complaint data from hospital emergency departments. Together, these methods will provide city public health agencies with a suite of tools and methods to improve the overall quality of population health and reduce health disparities.
Daniel B. Neill is an associate professor of Computer Science and Public Service at NYU’s Courant Institute Department of Computer Science and Robert F. Wagner Graduate School of Public Service, and Associate Professor of Urban Analytics at NYU’s Center for Urban Science and Progress, where he directs the Machine Learning for Good Laboratory. Dr. Neill’s research focuses on developing novel machine earning methods for social good, with applications ranging from medicine and public health to urban analytics and fairness in criminal justice. He received his M.Phil. from Cambridge University and his M.S. and Ph.D. in Computer Science from Carnegie Mellon University.
Dr. Neill’s research focuses on developing new methods for machine learning and event detection in massive and complex datasets, with applications ranging from medicine and public health to law enforcement and urban analytics. He works closely with organizations including public health, police departments, hospitals, and city leaders to create and deploy data-driven tools and systems to improve the quality of public health, safety, and security, for example, through the early detection of disease outbreaks and through predicting and preventing hot-spots of violent crime.