Author – Jessica Brassard
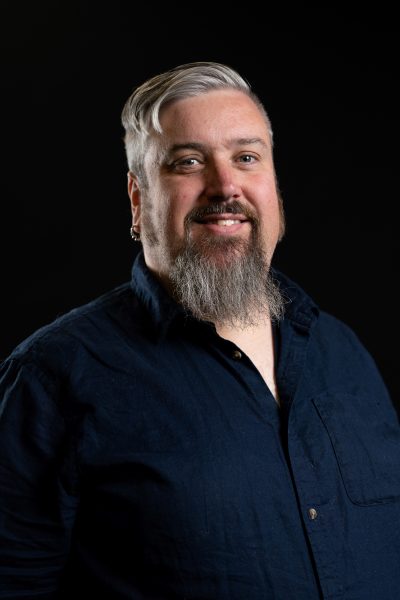
Introduction
In the realm of academia, researchers often find themselves enticed by the pursuit of fascinating and innovative problem spaces. Dr. Kevin Trewartha is an Associate Professor in Cognitive and Learning Sciences, on the executive committee of the Health Research Institute, and is a member of ICC’s Human Centered Computing Center. He is poised to embark on a journey that blends his expertise in cognitive neuroscience with big data computation. With exciting plans ahead during his Spring 2024 sabbatical, Trewartha aims to delve into the world of health computation in collaboration with researchers from Canada. This blog article offers a high-level view of Kevin’s pioneering ideas, particularly focusing on his pursuit of using computational approaches to identify pre-clinical behavior indicative of dementia, and the potential implications for Alzheimer’s Disease research.
The Quest for Early Indicators of Alzheimer’s Disease
Alzheimer’s Disease (AD) is a complex neurological condition characterized by a significant decline in cognitive function. Existing diagnostic tools mainly detect later-stage symptoms when individuals are already experiencing clinically-relevant levels of cognitive impairment. For many years, the field of Alzheimer’s research and clinical practice has been grappling with an important but challenging question: how do we identify individuals in the preclinical stage of AD? The problem stems from the fact that cognitive tests typically used to diagnose and track the progression of AD are not sufficiently sensitive to differences between normal age-related cognitive decline and cognitive impairment due to the earliest stages of AD.
Individuals, family members, caregivers, and clinicians alike are concerned with knowing whether a subjective cognitive complaint is related to natural aging or an early sign of dementia. We’d like to find ways to distinguish between the two with better accuracy.
Current approaches revolve around calculating composite scores from a battery of tests targeting various cognitive domains, including memory, cognitive control, and attention. A potential limitation of this approach is that it relies on those cognitive tests that are not individually sensitive to differences between normal aging and early AD, and composite scores may mask subtle variation in cognitive function necessary to identify the preclinical stage. Alternative approaches include searching for biomarkers or early disease stages using blood tests and neuroimaging, and the identification of functional decline using measures of cognitive dysfunction from instrumental activities of daily living and everyday cognition. It is clear that no single measure is sensitive to preclinical AD and it is necessary to find ways to identify behavioral and physiological patterns that better predict progression to AD.
Kevin’s vision revolves around identifying early warning signs using computational algorithms capable of discerning subtle patterns of differences between normal aging and preclinical AD in cognitive test performance, motor behavior, and neurophysiological function. His current research focuses on using motor tasks of varying complexity from reaching movements, to motor skill learning, to complex motor decision-making tasks to identify subtle changes in motor function that arise due to cognitive impairment in AD. Combining those behavioral measures with neurophysiological recordings in the form of EEG and EMG, and performance on more traditional cognitive tests produces massive amounts of data. The field typically reduces those data into a few key metrics rather than capitalizing on the full potential of underlying patterns that could distinguish normal aging from AD. By leveraging computational power, Kevin hopes to better analyze massive data sets that encompass cognitive scores, movement tracking, and high-level neurological information, including up to 64 channels of EEG data, and 10 channels of EMG data.
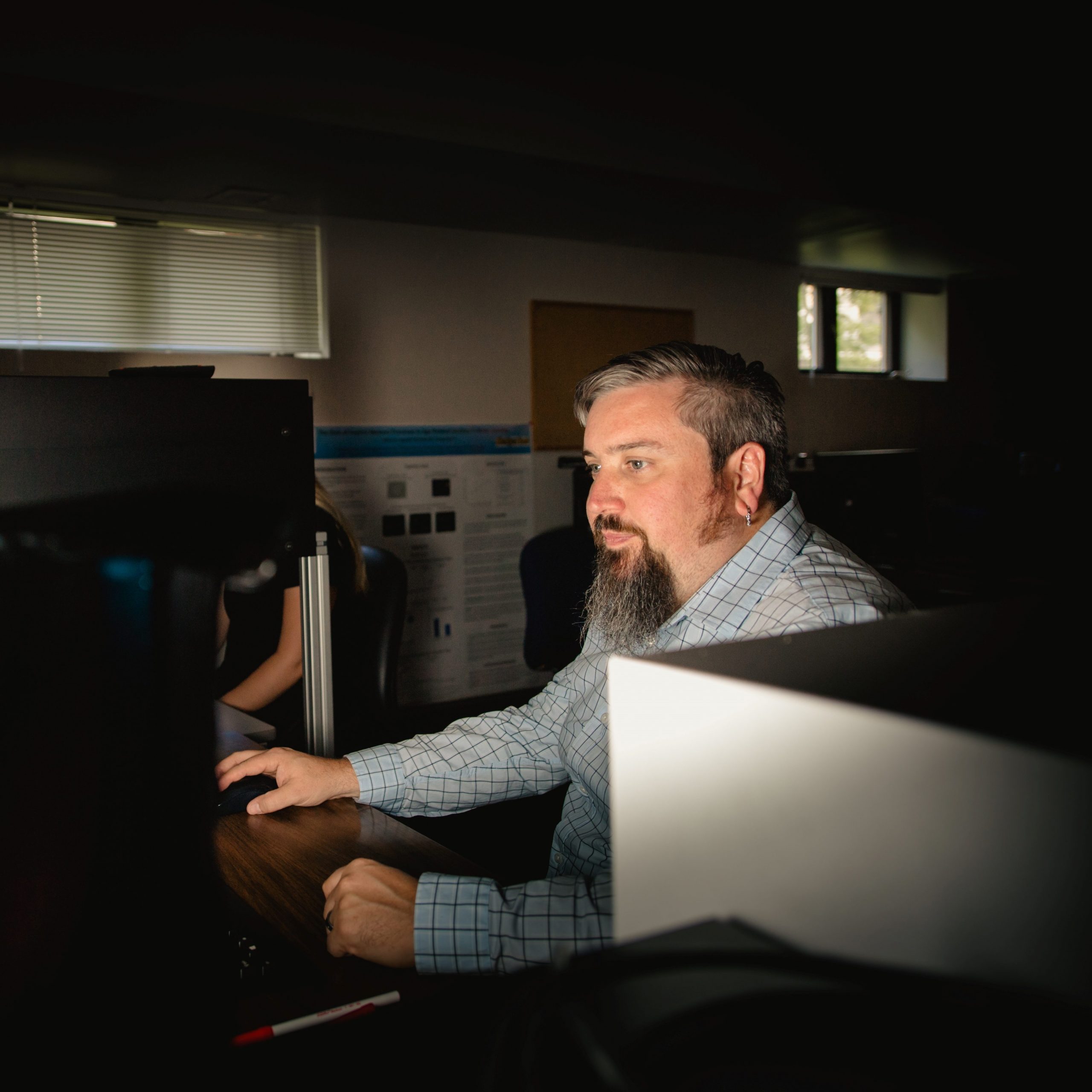
A Fusion of Health and Data Science
The overlap between cognitive science and computation is an exciting playground for innovation. While some cognitive scientists possess big data computational skills, many do not. Kevin sees this as a promising opportunity to leverage the collective strengths of cognitive and health scientists and data scientists to move research forward in dementia and related cognitive disorders.
“The specific data sets that we acquire could be complemented by additional measures such as other physiological metrics, biomarkers, and self-reported daily activities that could reveal a bigger picture pattern that distinguishes normal aging from dementia.”
He is interested in building collaborations with leaders in big data computation so together they can apply computational techniques to analyze vast and intricate datasets and uncover hidden patterns and insights that may hold the key to detecting pre-clinical signs of dementia. This innovative approach could potentially open new doors for early intervention and the development of strategies to slow the disease’s progress.
Trends in Sponsor Funding
Funding agencies have been developing more programs for researchers to make progress in this area. Funding agencies like the National Institutes of Health (NIH) and the Alzheimer’s Association are actively encouraging studies that target more complex behavioral tasks and more thorough analyses of neurophysiological datasets to identify meaningful patterns. With the availability of massive datasets related to batteries of cognitive tasks, kinematic patterns during motor tasks, neurophysiological recordings, neuroimaging, and biomarkers, the field is poised for substantial growth in our understanding of the holistic pattern behavioral neuropathology in the preclinical stages of AD.
Conclusion
By combining Kevin’s expertise with collaborators’ computational skills, he seeks to better understand pre-clinical dementia indicators and lay the groundwork for transformative research. This pursuit has the potential to revolutionize the way we approach Alzheimer’s Disease and other cognitive disorders, ultimately leading to improved patient outcomes and a deeper understanding of the human mind.
I read this blog and very impressive and motivational article.