The Colleges of Computing and Engineering invite the campus community to a lecture by faculty candidate Hongyu An on Wednesday, February 12, at 3:00 p.m. in Chem Sci 101. Hongyu’s talk is titled, “Brain on a Chip: Designing Self-learning and Low-power Neuromorphic Systems.”
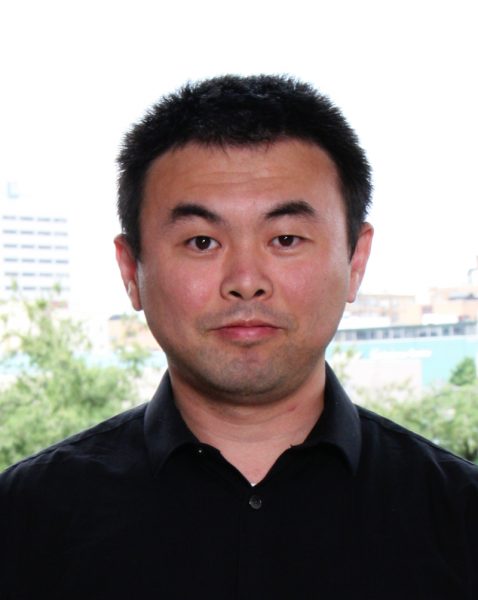
Hongyu is a doctoral candidate in the Bradley Department of Electrical and Computer Engineering, Virginia Polytechnic Institute and State University (Virginia Tech). He received B.S. and M.S. degrees in electrical engineering at Shenyang University of Technology, Shenyang, China, and the Missouri University of Science and Technology, Rolla, Mo., respectively.
In 2019, Hongyu was awarded the Paul E. Torgersen Research Excellence Award and a fellowship from the Advanced Short-Term Research Opportunity Program at Oak Ridge National Laboratory. In 2017, he was awarded an NSF Student Travel Fellowship Award, and a paper authored by Hongyu was nominated as best paper in the IEEE International Symposium on Quality Electronic Design (ISQED).
Hongyu’s research interests include neuromorphic and brain-inspired computing, energy-efficient neuromorphic electronic circuit design for Artificial Intelligence, three-dimensional integrated circuit (3D-IC) design, and emerging nanoscale device design.
His research aims to build a self-learning, low-power neuromorphic system. Inspired by the learning mechanism of the human brain, Hongyu proposed and realized an Associative Memory Learning through neuromorphic circuits and memristors. The proposed learning method correlates two concurrent visual and auditory information together through Artificial Neural Networks.
Lecture Abstract: How can a silicon brain in a chip be built with self-learning capability? What are the challenges for neural network-based artificial intelligence in the next decade, and how can those challenges be solved?
In order to answer these questions, Hongyu introduces a cutting-edge research topic: Brain-inspired Computing. Also called neuromorphic computing, Brain-inspired Computing aims to physically reproduce the brain’s structure in a silicon chip to resolve critical challenges in deep learning deployment.
In his talk, Hongyu will explore the underlying biological mechanism of associative memory learning, novel non-von Neumann computer architectures, and circuit implementations with transistors and memristors.
A widespread self-learning method in animals, associative memory enables the nervous system to remember the relationship between two concurrent events. Rebuilding associative memory is significant, both to reveal a way of designing a brain-like self-learning neuromorphic system, and to explore a method of comprehending the function of the human brain.
Hingyu is a reviewer for several top-tier conferences and journals, including IEEE Transactions on Neural Networks and Learning Systems (TNNLS), IEEE Transactions on Circuits and System I: Regular Papers (TCAS-1),Design Automation Conference (DAC). Design, Automation and Test in Europe Conference and Exhibition (DATE), International Symposium on Circuits and Systems (ISCAS).