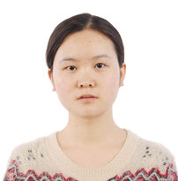
Jingjing Yao, a PhD candidate in computer engineering at New Jersey Institute of Technology, will present a talk on Tuesday, May 11, at 3:00 p.m.
Her talk is titled, “Intelligent and Secure Fog-Aided Internet of Drones.”
Yao’s research interests include Internet of Things (IoT), Internet of Drones (IoD), Deep Reinforcement Learning, Federated Learning, Cybersecurity, Mobile Edge Computing/Caching, and Energy Harvesting.
Talk Title
Intelligent and Secure Fog-Aided Internet of Drones
Talk Abstract
Internet of drones (IoD), which deploys several drones in the air to collect ground information and send them to the IoD gateway for further processing, can be applied in traffic surveillance and disaster rescue. Fog-aided IoD provisions future events prediction and image classification by machine learning technologies, where massive training data are collected by drones and analyzed in the fog node. However, the performance of IoD is greatly affected by drones’ battery capacities. Also, aggregating all data in the fog node may incur huge network traffic and drone data privacy leakage.
The speaker will share her vision and research to address these two challenges. In this talk, the speaker utilizes energy harvesting technology to charge drone batteries and investigate wireless power control to adjust the drone wireless transmission power to reduce drone energy consumption. The joint optimization of power control and energy harvesting scheduling is investigated in time-varying IoD networks to minimize the long-term average system energy cost constrained by the drone battery capacities and quality of service (QoS) requirements.
A modified actor-critic deep reinforcement learning algorithm is designed to address the joint optimization problem in time-varying IoD networks. To prevent the privacy leakage of IoD, the speaker utilizes federated learning (FL) by performing local training in drones and sharing all training model parameters in the fog node without uploading drone raw data.
However, drone privacy can still be divulged to ground eavesdroppers by wiretapping and analyzing uploaded parameters during the FL training process. The power control problem is hence investigated to maximize the FL system security rate constrained by drone battery capacities and the FL training time requirement. An algorithm with low computational complexity is then designed to tackle the security rate maximization problem and its performance is demonstrated by extensive simulations.
Biography
Jingjing Yao is currently a Ph.D. candidate in Computer Engineering with the Department of Electrical and Computer Engineering at the New Jersey Institute of Technology (NJIT). She will receive her Ph.D. degree from NJIT in May 2021. She received the M.E. degree in Information and Communication Engineering from the University of Science and Technology of China (USTC), and the B.E. degree in Information and Communication Engineering from the Dalian University of Technology (DUT).
She has published 13 first-author journal articles and seven first-author conference papers. Her research interests include Internet of Things (IoT), Internet of Drones (IoD), Deep Reinforcement Learning, Federated Learning, Cybersecurity, Mobile Edge Computing/Caching, and Energy Harvesting.