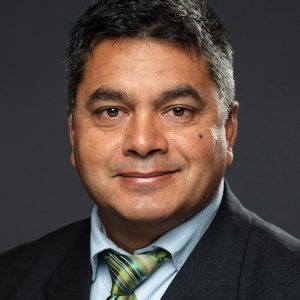
A book edited by Dukka KC, Computer Science, has been published by Springer Nature. The book, Computational Methods for Predicting Post-Translational Modification Sites, is part of the Springer book series, Methods in Molecular Biology. Learn more and purchase the book here.
The book aims to be a useful guide for researchers who are interested in the field of PTM site prediction. The volume describes computational approaches to predict multitudes of PTM sites. Chapters describe in-depth approaches on algorithms, state-of-the-art Deep Learning-based approaches, hand-crafted features, physico-chemical based features, issues related to obtaining negative training, sequence-based features, and structure-based features.
Written in the format of the highly successful Methods in Molecular Biology series, each chapter includes an introduction to the topic, lists necessary materials and reagents, includes tips on troubleshooting and known pitfalls, and step-by-step, readily reproducible protocols.
Dukka KC is associate dean for research, College of Computing; associate professor, Computer Science; and associate director for the Institute of Computing and Cybersystems (ICC). His research interests include bioinformatics, data science, machine learning/deep learning, and high-performance computing.
Publication Abstract
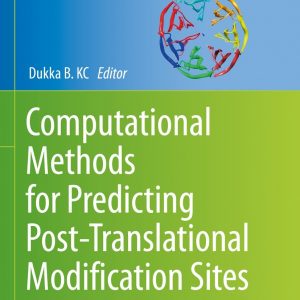
Post-translational modification (PTM) is a ubiquitous phenomenon in both eukaryotes and prokaryotes, which gives rise to enormous proteomic diversity. PTM mostly comes in two flavors: covalent modification to polypeptide chain and proteolytic cleavage. Understanding and characterization of PTM is a fundamental step toward understanding the underpinning of biology. Recent advances in experimental approaches, mainly mass-spectrometry-based approaches, have immensely helped in obtaining and characterizing PTMs. However, experimental approaches are not enough to understand and characterize more than 450 different types of PTMs and complementary computational approaches are becoming popular. With the accumulation of a large number of high-throughput datasets that can be used to develop various computational approaches, complementary computational approaches are becoming popular and increasingly critical in unraveling post-translational modifications. Needless to say, understanding and characterization of PTM is a fundamental step toward understanding the functions of a proteome.
In this book, well-established computational tools for various types of post-translational modifications are introduced. In addition, we have tried our best to cover various aspects of computational post-translational modification prediction: prediction of PTM sites, prediction/analysis of functional PTM sites, visualization of PTM, development of databases for PTMs., prediction/analysis of PTM cross-talk, tools for proteolytic cleavage among others.
The book is organized taking into consideration the steps for developing a computation tool, especially machine learning tools. The book discusses these routine tasks in any Machine Learning-based approach: i) creation of the benchmark dataset (to train and test), ii) extraction of features or encoding of the input protein sequence, and iii) develop the appropriate machine learning architecture. Additionally, as the field of computational prediction of PTM has also witnessed the development of various algorithms including a plethora of Deep Learning- (DL)-based approaches. In this book, we also discuss recent DL-based approaches in the field of PTM site prediction. In addition to in-depth approaches on algorithms, state-of-the art Deep learning architecture, we also describe feature encoding, novelty of the approaches, and availability of the tools/approaches. Finally, we provide an outlook and possible future research directions for PTM prediction.