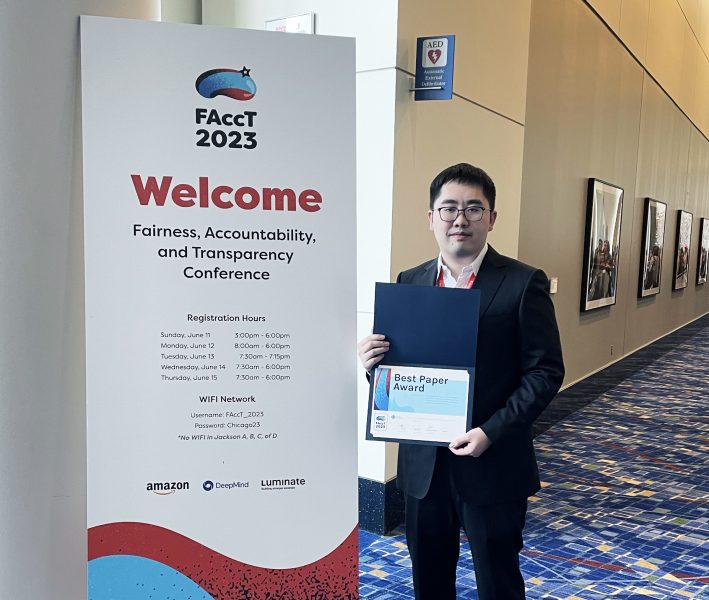
A paper co-authored by graduate student Zichong Wang, Computer Science, has been accepted for presentation at the European Conference on Machine Learning and Principles and Practice of Knowledge Discovery in Databases (ECML/PKDD 2023), which takes place September 18-22, 2023, in Turin, Italy.
Wang is advised by Assistant Professor Wenbin Zhang, Computer Science.
The paper is titled, “F$G^2$AN: Fairness-aware Graph Generative Adversarial Networks.” Wang’s co-authors are Zhang and Associate Professor Charles Wallace, Computer Science.
Wang’s paper was one of 199 (24%) selected for presentation at the conference from a pool of 830 submissions.
Paper Abstract: Graph generation models have gained increasing popularity and success across various domains. However, most research in this area has concentrated on enhancing performance, with the issue of fairness remaining largely unexplored. Existing graph generation models prioritize minimizing graph reconstruction’s expected loss, which can result in representational disparities in the generated graphs that unfairly impact marginalized groups. This paper addresses this socially sensitive issue by conducting the first comprehensive investigation of fair graph generation models by identifying the root causes of representational disparities, and proposing a novel framework that ensures consistent and equitable representation across all groups. Additionally, a suite of fairness metrics has been developed to evaluate bias in graph generation models, standardizing fair graph generation research. Through extensive experiments on five real-world datasets, the proposed framework is demonstrated to outperform existing benchmarks in terms of graph fairness while maintaining competitive prediction performance.