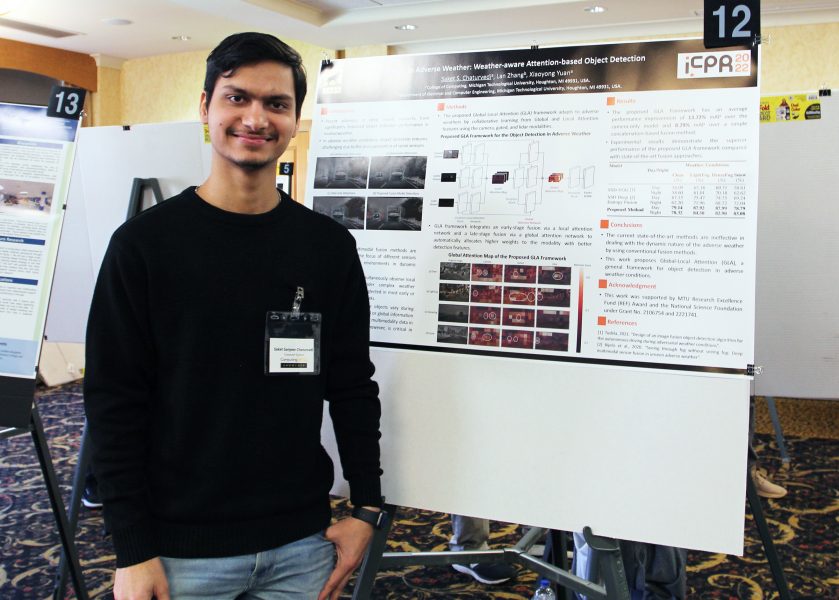
PhD student Saket Chaturvedi will present his dissertation proposal on Tuesday, October 24, 2023, from 8:30-10:30 am in Rekhi 101 and via Zoom online meeting. The title of the proposal is, “Securing Autonomous Driving: Backdoor Attacks on Multimodal Fusion Methods.”
Chaturvedi is advised by Brian (Xiaoyong) Yuan.
Proposal Title
Securing Autonomous Driving: Backdoor Attacks on Multimodal Fusion Methods
Proposal Abstract
3D object detection has evolved into a fundamental component in many state-of-the-art autonomous driving systems. The effectiveness of 3D object detection relies significantly on the integration of multiple sensors, including cameras and LiDAR. In this context, multimodal fusion techniques are extensively employed to enhance object detection performance. However, despite the critical role of multimodal fusion in advancing perception systems, its security implications have received limited attention. Concurrently, while deep neural networks have made significant strides in 3D object detection, vulnerabilities to backdoor attacks have been identified.
This research is motivated by the imperative to investigate the security aspects of Multimodal Fusion methods, with a particular focus on their susceptibility to Backdoor Attacks. Our primary objective is to assess the impact of backdoor attacks on multimodal fusion methods employed in 3D object detection systems. In particular, we plan to scrutinize the robustness of 3D object detection by exploring a novel backdoor attack vector involving 2D cameras, aiming to uncover the latent potential for camera signals to disrupt this critical 3D Fusion process. This research endeavor aims not only to uncover potential vulnerabilities but also to enhance the robustness of 3D object detection systems against backdoor threats in the context of evolving multi-modal fusion systems. By comprehensively evaluating the security of multimodal fusion methods in 3D object detection, we contribute to the development of more resilient and dependable autonomous driving systems.