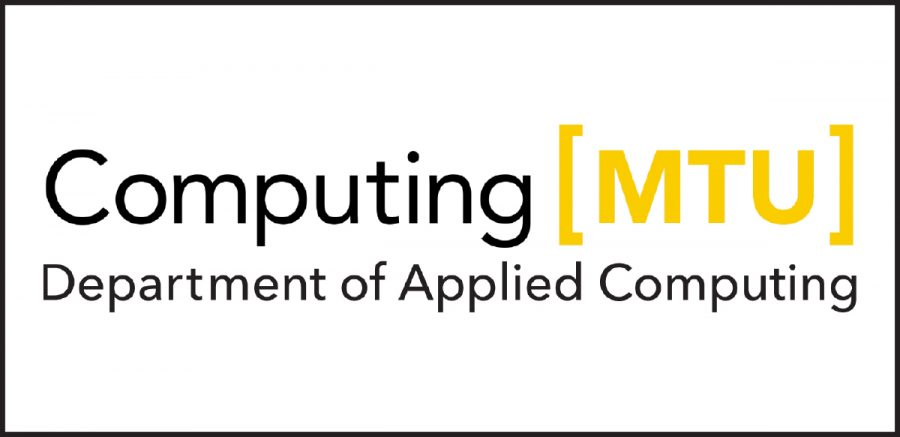
Department of Applied Computing tenure-track faculty candidate Keith Feldman will give a research presentation on Friday, March 15, 2024, at 2 pm in Rekhi 214. The title of Feldman’s presentation is, “Beyond Modeling: Contextualizing Data and Improving Patient Representations in the Context of Learning Health Systems.”
Talk Abstract
In line with the values of P4 (predictive, preventive, participatory, and personalized) medicine, healthcare today has continued to provide increasingly unique care for each patient. While this form of individualized care has been shown to improve outcomes, there exists a fundamental conflict between completely personalized medicine and the success of machine learning and statistical tools that have excelled in extracting patterns from large repositories of data. There exists a need to reframe the expectations of computational tools from simply utilizing data for prediction. Expanding these techniques to those that can draw insight and provide useful context from the diverse body of the data we collect through routine care. This talk will highlight ongoing research to improve the way computational tools leverage representations of patient data to create a more complete view of a given individual’s health over time, as well as how contextualization of such information can not only aid in current clinical processes, but advance them.
Candidate Bio
Dr. Feldman is a computer scientist by training who has spent his graduate, postdoctoral, and early faculty career developing a portfolio of research in the area of computational health, applying machine learning and data science techniques to problems across the healthcare domain. Tied to the potential of these techniques to capture variability between patient conditions and outcomes, his work has identified patient subtypes, evidenced-based risk measures, and treatment patterns tied to the quality and effectiveness of care. Working closely with multidisciplinary teams, this work is fundamentally motivated by the notion of augmentation, not automation. Rather than utilizing computation to replicate healthcare decisions, he aims to augment the existing skillsets of those engaged in healthcare. Broadly seeking to determine what information, if available, would improve decisions relevant to their role. His work is funded by the NIH, AHA, Frontiers CTSI and generous philanthropic gifts.