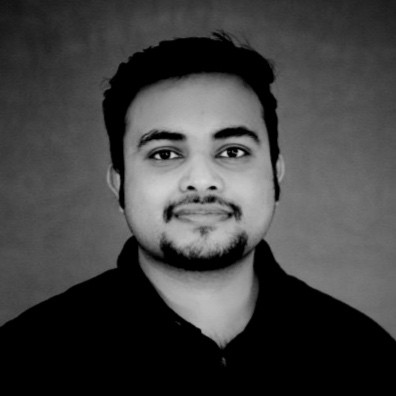
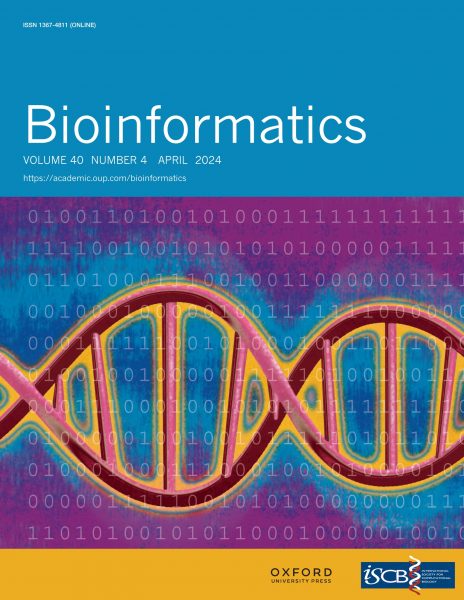
A paper by graduate student Pawel Pratyush, PhD in Computer Science, has been published in Bioinformatics, a publication of Oxford University Press and a top journal in the bioinformatics field.
The title of the paper is, “LMCrot: An enhanced protein crotonylation site predictor by leveraging an interpretable window-level embedding from a transformer-based protein language model.”
Pratyush’s co-authors are Soufia Bahmani (PhD student, Computer Science), Suresh Pokharel (PhD student, Computer Science), Hamid D Ismail (postdoctoral scholar, Computer Science), and Dukka B KC (professor, Computer Science).
Paper Abstract
Motivation
Recent advancements in natural language processing have highlighted the effectiveness of global contextualized representations from Protein Language Models (pLMs) in numerous downstream tasks. Nonetheless, strategies to encode the site-of-interest leveraging pLMs for per-residue prediction tasks, such as crotonylation (Kcr) prediction, remain largely uncharted.’
Results
Herein, we adopt a range of approaches for utilizing pLMs by experimenting with different input sequence types (full-length protein sequence versus window sequence), assessing the implications of utilizing per-residue embedding of the site-of-interest as well as embeddings of window residues centered around it. Building upon these insights, we developed a novel residual ConvBiLSTM network designed to process window-level embeddings of the site-of-interest generated by the ProtT5-XL-UniRef50 pLM using full-length sequences as input. This model, termed T5ResConvBiLSTM, surpasses existing state-of-the-art Kcr predictors in performance across three diverse datasets. To validate our approach of utilizing full sequence-based window-level embeddings, we also delved into the interpretability of ProtT5-derived embedding tensors in two ways: firstly, by scrutinizing the attention weights obtained from the transformer’s encoder block; and secondly, by computing SHAP values for these tensors, providing a model-agnostic interpretation of the prediction results. Additionally, we enhance the latent representation of ProtT5 by incorporating two additional local representations, one derived from amino acid properties and the other from supervised embedding layer, through an intermediate-fusion stacked generalization approach, using an n-mer window sequence (or, peptide fragment). The resultant stacked model, dubbed LMCrot, exhibits a more pronounced improvement in predictive performance across the tested datasets.
The paper is publicly available at https://github.com/KCLabMTU/LMCrot.