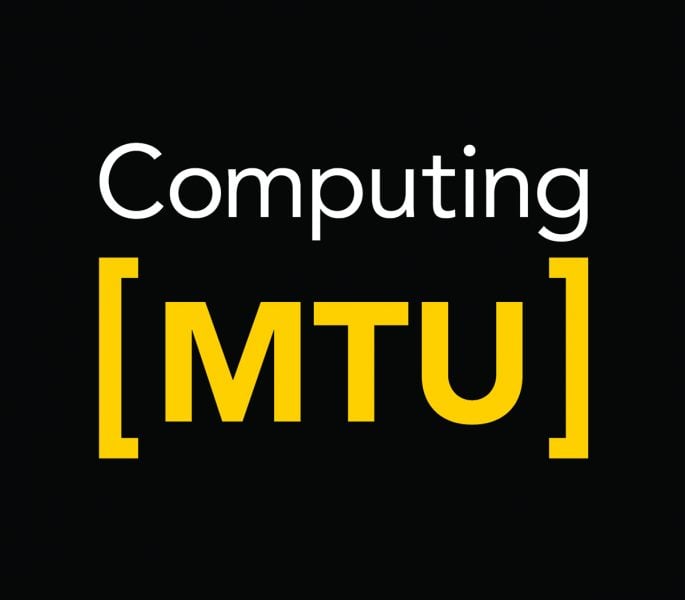
Department of Applied Computing PhD student Ali Awad, Computational Science and Engineering, will present a defense proposal on Tuesday, August 13, 2024, at 10 am in Rekhi 101 and via Zoom online meeting.
Please note that the date and time of this event has been changed from its original date and time of August 7 at 11 am. The Zoom link has not changed.
The topic of Awad’s proposal is, “Enhancing Underwater Image Quality for Effective Object Detection.”
Awad is advised by Ashraf Saleem.
Proposal Abstract
Underwater imagery often suffers from severe degradation that results in low visual quality and object detection performance. Current underwater datasets are limited in terms of the number of images, classes and scenes compared to in-air datasets. In addition, the annotation accuracy of underwater dataset is affected by the severe degradation and low-light conditions causing human annotator to mislabel some objects. Researchers developed a few metrics to assess the quality of underwater images, however, those metrics are sensitive to noise and do not align with human perception of quality on numerous occasions. Image enhancement often has a negative impact on underwater object detection which motivated researchers to address the combined problem of enhancement and detection in effort to produce visually pleasing images and high detection performance. However, those studies are still scarce and limited. This work aims to evaluate state-of-the-art image enhancement models, investigate their impact on underwater object detection, and explore their potential to improve detection performance. To this end, we studied different image enhancements algorithms covering all major enhancement categories and developed our own. In addition, we used publicly available benchmark underwater datasets and collected our own. Following this, we conducted qualitative and quantitative analyses on the enhanced images and developed a quality index (Q-index) to compare the quality distribution of the original and enhanced images. Subsequently, we compared the performance of several detection models that are separately trained and tested on the original and enhanced image sets. Then, we performed a correlation study to examine the relationship between enhancement metrics and detection performance. We also analyzed the inference results from the trained detectors and uncovered cases where enhancement, in contrast to previous studies, improves detection performance as well as cases where detection performance was affected by ground truth errors. This study indicates that enhancement has the potential to increase detection accuracy due to a reduced gap between low and high-quality images. In addition, we reveal that current underwater object detection datasets could be suffering from human annotation errors due to limited visual quality resulting in unreliable conclusions. This underscores the necessity for a thorough reassessment of the enhancement-detection framework.