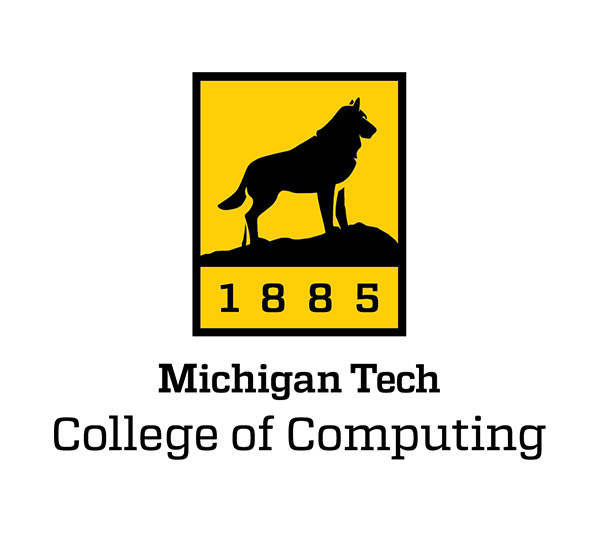
Are you interested in a faculty position with the new Michigan Tech College of Computing? Do you know someone who is?
Michigan Technological University’s College of Computing invites applications for two (2) assistant, associate, or full professor positions to start in August 2021.
Areas of particular interest include cybersecurity, artificial intelligence/machine learning, and data science; exceptional candidates in other areas of computing will also be considered.
Successful candidates will demonstrate a passion for their research, an enthusiasm for undergraduate and graduate education, and a strong commitment to cultivating diverse and inclusive learning environments.
View the positions description and apply here: https://www.employment.mtu.edu/cw/en-us/job/492473
Review of applications will begin immediately and continue until the position is filled. To learn more about this opportunity, please visit https://www.mtu.edu/computing/about/employment/ or contact the search chair, Dr. Timothy Havens, at thavens@mtu.edu. Applications received by March 1, 2020 will receive full consideration.
Michigan Tech is building a culturally diverse faculty committed to teaching and working in a multicultural environment and strongly encourages applications from all individuals. We are an ADVANCE Institution having received three National Science Foundation grants in support of efforts to increase diversity, inclusion, and the participation and advancement of women and underrepresented individuals in STEM.
Michigan Tech actively supports dual-career partners to retain a quality workforce; we offer career exploration advice and assistance finding positions at the University and in the local community. Please visit https://www.mtu.edu/provost/programs/partner-engagement for more information.
An applicant must have earned a Ph.D. degree in Computer Science, Computer Engineering, Computing, or a closely related area. Michigan Tech places a strong emphasis on balancing cutting-edge research with effective teaching, outreach, and service. Candidates for these positions are expected to demonstrate potential for excellence in independent research, excellence in teaching, and the ability to contribute service to their department and profession. Salary is negotiable depending upon qualifications.
Michigan Tech is an internationally renowned doctoral research university with 7,100 students and 400 faculty located in Houghton, Michigan, in the scenic Upper Peninsula on the south shore of Lake Superior. The area provides a unique setting where natural beauty, culture, education, and a diversity of residents from around the world come together to share superb living and learning experiences.
The College of Computing has 36 faculty members, 650 undergraduate students in five degree programs (Computer Science, Computer Network and System Administration, Cybersecurity, Electrical Engineering Technology, Mechatronics, and Software Engineering) and 90 graduate students in four MS degree programs (Computer Science, Cybersecurity, Data Science, Health Informatics, and Mechatronics) and Ph.D. degree programs in Computer Science and Computational Science and Engineering.