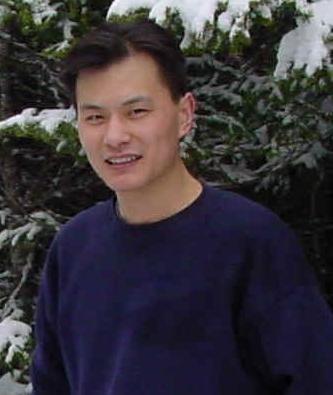
The Department of Computer Science will present a lecture by Dr. Qun Li on Friday, April 23, 2021, at 3:00 p.m. Dr. Li is a professor in the computer science department at William and Mary university. The title of his lecture is, “Byzantine Fault Tolerant Distributed Machine Learning.”
Lecture Title
Byzantine Fault Tolerant Distributed Machine Learning
Lecture Abstract
Training a deep learning network requires a large amount of data and a lot of computational resources. As a result, more and more deep neural network training implementations in industry have been distributed on many machines. They can also preserve the privacy of the data collected and stored locally, as in Federated Deep Learning.
It is possible for an adversary to launch Byzantine attacks to a distributed or federated deep neural network training. That is, some participating machines may behave arbitrarily or maliciously to deflect the training process. In this talk, I will discuss our recent results on how to make distributed and federated neural network training resilient to Byzantine attacks. I will first show how to defend against Byzantine attacks in a distributed stochastic gradient descent (SGD) algorithm, which is the core of distributed neural network training. Then I will show how we can defend against Byzantine attacks in Federated Learning, which is quite different from distributed training.