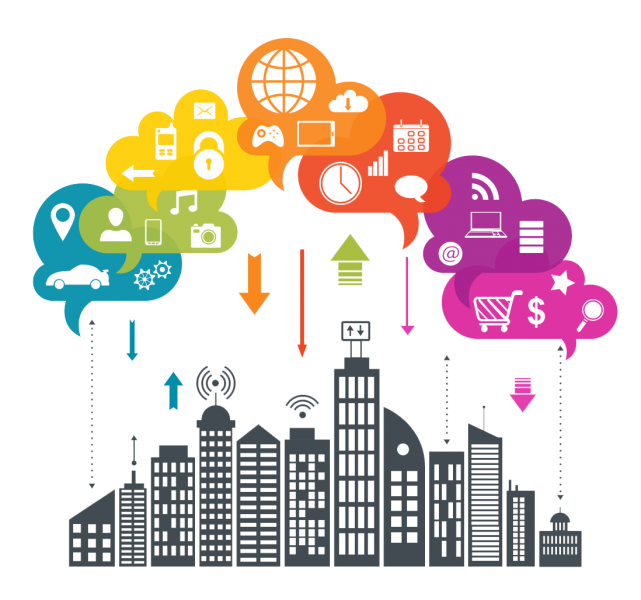
The Colleges of Computing and Engineering invite the campus community to a lecture by faculty candidate Lan Zhang on Wednesday, February 5, 2020, at 3:00 p.m., in Chem. Sci. 101. Zhang’s lecture is titled, “Machine Learning Enabled Better Cyber-Physical Systems: A Case Study on Better Networking for Connected Vehicles.”
Bio: Lan Zhang is a Ph.D. candidate in the Department of Electrical and Computer Engineering at the University of Florida. She received the B.Eng. and M.S. degrees in telecommunication engineering from the University of Electronic Science and Technology of China, in 2013 and 2016, respectively. Zhang’s research interest spans across the fields of big data, cyber-physical systems, machine learning, wireless communications, and cybersecurity. She has published 15 technical papers in top-tier journals and conference venues, such as IEEE Transactions on Vehicular Technology, Proceedings of the IEEE, and IEEE Transactions on Wireless Communication.
Zhang has served as a technical program committee (TPC) member for several high-quality conferences, such as the 2020 IEEE INFOCOM poster/demo section and the 2018 International Conference on Computing, Networking and Communications. She also serves as reviewer for several leading journals, such as IEEE Transactions on Communications, IEEE Transactions on Vehicular Technology, IEEE Transactions on Mobile Computing, and IEEE Transactions on Wireless Computing. Zhang was the speaker at several flagship celebrations and conferences, such as IEEE Global Communications Conference 19, Grace Hopper Celebration 19, and the IEEE International Conference on Communications 19.
Lecture Abstract: With the recent success of big data analytics, machine learning is being used in various Cyber-Physical Systems (CPS) applications, such as smart transportation, smart healthcare, and industrial automation. As a highly interdisciplinary field, the CPS applications require the machine learning-enabled wireless communication strategies to facilitate information exchanges, and meanwhile call for secure and private learning pipelines to manage information exchanges.
In her talk, Zhang focuses on connected vehicles, aiming at supporting the demand for multi-Gbps sensory data exchanges through millimeter-wave bands for enhancing (semi)-autonomous driving. Unlike most traditional networking analysis that manipulates end devices to adapt to the transmission environments, i.e., fight against any transmission obstacles, we propose an innovative idea to proactively manipulate, reconfigure, and augment the transmission environments for better communications.
Without damaging the aesthetic nature of environments, we deploy multiple small-piece controllable reflecting surfaces, and adaptively manipulate the angle of the used reflecting surfaces to address the vulnerability of blockages in mmWave vehicular communications by creating alternative indirect line-of-sight connections. To autonomously and efficiently augment the highly dynamic vehicular environments in real-time, deep reinforcement learning techniques are implemented. Effectiveness of our proposal is showcased on the traffic at the City of Luxembourg using a traffic simulation toolkit, Simulation of Urban MObility (SUMO).