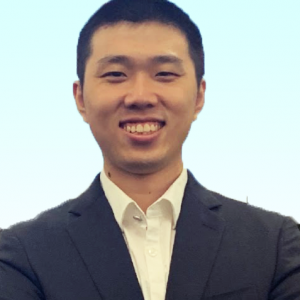
The Colleges of Computing and Engineering invite the campus community to a lecture by faculty candidate Xiaoyong (Brian) Yuan on Wednesday, February 26, 2020, at 3:00 p.m. in Chem Sci 101. Yuan’s talk is titled, “Secure and Privacy: Preserving Machine Learning, A Case Study on Model Stealing Attacks Against Deep Learning.”
Brian Yuan is a computer science Ph.D. candidate at the University of Florida. He received an M.E. degree in computer engineering from Peking University in 2015, and a B.S. degree in mathematics from Fudan University in 2012. His research interests span the fields of deep learning, machine learning, security and privacy, and cloud computing.
In his talk, Yuan will provide an overview of security and privacy issues in deep learning, then focus on his recent research on a data-agnostic model stealing attack against deep learning. He will conclude with a discussion of some future research directions to address security and privacy concerns in deep learning and potential countermeasures.
Due to recent breakthroughs, machine learning, especially deep learning, is pervasively serving areas such as autonomous driving, game playing, and virtual assistants. Recently however, significant security and privacy concerns have been raised in deploying deep learning algorithms.
On one hand, deep learning algorithms are fragile and easily fooled by attacks. For example, an imperceptible perturbation on a traffic sign can mislead the autonomous driving systems. On the other hand, with the increasing use of deep learning in personalization, virtual assistants, and healthcare, deep learning models may expose users’ sensitive and confidential information.
With important business value, deep learning models have become essential components in various commercialized machine learning services, such as Machine Learning as a Service (MLaaS). Model stealing attacks aim to extract a functionally equivalent copy of deep learning models and cause a breach of confidentiality and integrity of deep learning algorithms. Most existing model stealing attacks require private training data or auxiliary data from service providers, which significantly limits the attacking impact and practicality. Yuan proposes a much more practical attack without the hurdle of training data, and its effectiveness will be showcased in several widely used datasets.
Yuan has published 17 papers in top-tier journals and conferences, such as IEEE Transactions on Neural Networks and Learning Systems (TNNLS) and the AAAI Conference on Artificial Intelligence (AAAI). He has served as reviewer for several leading journals and conferences, such as IEEE Transactions on Neural Networks and Learning Systems (TNNLS), International Conference on Learning Representations (ICLR), IEEE Transactions on Dependable and Secure Computing (TDSC), and IEEE Transactions on Parallel and Distributed Systems (TPDS).
Read the blog post here: https://blogs.mtu.edu/computing/2020/02/12/faculty-candidate-brian-yaun-to-present-lecture/