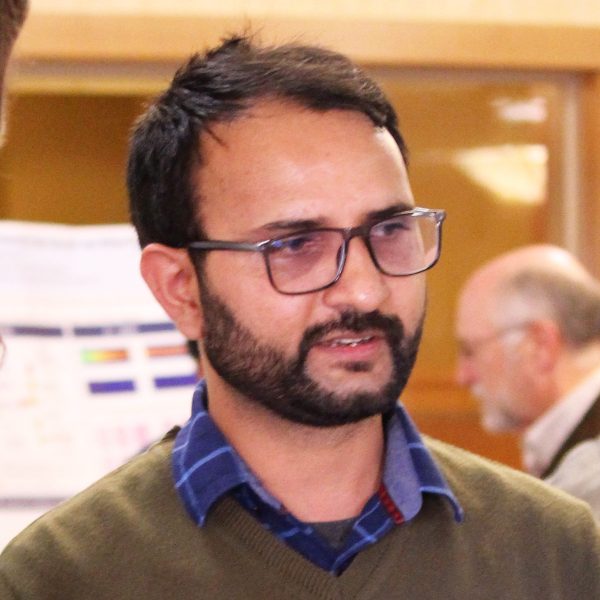
Graduate student Suresh Pokharel, Computer Science, will present his PhD dissertation proposal on Friday, November 3, 2023, from 2-4 pm in Rekhi 101 and via Zoom online meeting. The title of Pokharel’s proposal is, “Enhancing PTM Prediction: Leveraging Protein Language Model Embeddings for Accurate Insights.”
Pokharel is advised by Dukka B. KC.
Proposal Title
Enhancing PTM Prediction: Leveraging Protein Language Model Embeddings for Accurate Insights
Proposal Abstract
Post-translational modifications (PTMs) are chemical changes that occur in proteins after they are synthesized from mRNA during translation. These modifications are vital for ensuring the correct structure and function of proteins, and they play a pivotal role in regulating numerous cellular processes. Given their critical role in governing cellular functions, such as gene expression and protein degradation, any dysregulation in PTMs can lead to various diseases and disorders. Therefore, a comprehensive understanding of post-translational modifications and detecting their presence is essential for unraveling the intricacies of cellular biology and molecular mechanisms. Among the various types of post-translational modifications (PTMs), this study investigates two highly prominent and extensively studied ones: succinylation and O-GlcNAcylation. Our preliminary work focuses predominantly on leveraging various natural language programming (NLP) techniques, including various sequence-based protein language models (pLMs), where we developed predictive models capable of accurately predicting the occurrence of these PTMs. This proposal aims to enhance the robustness of predictive models through two key approaches. Firstly, by integrating three-dimensional protein structure information to enrich the existing sequence-based feature information. Secondly, we further propose fine-tuning the generic large protein language models to effectively adapt them to our limited task-specific data. By developing a robust framework for PTMs (Succinylation and O-GlcNAc) prediction, this research holds the promise of advancing our understanding of biology, improving healthcare outcomes, and accelerating drug discovery by providing better insights into the regulatory mechanisms of protein modifications.