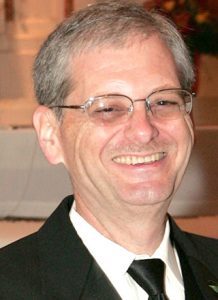
Dr. James Keller, recently retired Curators’ Distinguished Professor in the EE/CS department at University of Missouri, Columbia, will present his lecture, “Soft Streaming Classification,” on Friday, October 30, 2020, at 3:00 p.m., via Zoom online meeting.
The talk is an Institute of Computing and Cybersystems’ (ICC) Distinguished Lecture Series event.
Join the meeting here.
A Life Fellow of the Institute of Electrical and Electronics Engineers (IEEE), Keller recently received the IEEE Frank Rosenblatt Award for his “fundamental work on fuzzy pattern recognition, fuzzy clustering, and fuzzy technologies in computer vision.” He holds a number of additional professional and academic honors and awards.
Lecture Abstract
As the volume and variety of temporally acquired data continues to grow, increased attention is being paid to streaming analysis of that data. Think of a drone flying over unknown terrain looking for specific objects which may present differently in different environments. Understanding the evolving environments is a critical component of a recognition system.
With the explosion of ubiquitous continuous sensing (something Lotfi Zadeh predicted as one of the pillars of Recognition Technology in the late 1990s), this on-line streaming analysis is normally cast as a clustering problem. However, examining most streaming clustering algorithms leads to the understanding that they are actually incremental classification models.
These approaches model existing and newly discovered structures via summary information that we call footprints. Incoming data is routinely assigned crisp labels (into one of the structures) and that structure’s footprints are incrementally updated; the data is not saved for iterative assignments.
The three underlying tenets of static clustering:
- Do you believe there are any clusters in your data?
- If so, can you come up with a technique to find the natural grouping of your data?
- Are the clusters you found good groupings of the data?
These questions do not directly apply to the streaming case. What takes their place in this new frontier?
In this talk, I will provide some thoughts on what questions can substitute for the Big 3, but then focus on a new approach to streaming classification, directly acknowledging the real identity of this enterprise. Because the goal is truly classification, there is no reason that these assignments need to be crisp.
With my friends, I propose a new streaming classification algorithm, called StreamSoNG, that uses Neural Gas prototypes as footprints and produces a possibilistic label vector (typicalities) for each incoming vector. These typicalities are generated by a modified possibilistic k-nearest neighbor algorithm.
Our method is inspired by, and uses components of, a method that we introduced under the nomenclature of streaming clustering to discover underlying structures as they evolve. I will describe the various ingredients of StreamSoNG and demonstrate the resulting algorithm on synthetic and real datasets.
David Watkins (CEE/SFI) is the principal investigator on a project that has received a $190,764 research and development grant from the National Science Foundation (NSF).
The project is titled “RAPID: COVID-19, Consumption, and Multi-dimensional Analysis of Risk (C-CAR)“. Chelsea Schelly (SS/SFI), Robert Handler (ChE/SFI) and Charles Wallace (CS/SFI) are co-PIs on this one-year project.
Extract
The COVID-19 pandemic has transformed household dynamics and dramatically changed food, energy, and water consumption within the home. Stay-at-home orders and social distancing has caused U.S. households to shift to working and schooling from home, curtail outside activities, and stop eating in restaurants. Furthermore, as many households face job loss and increasing home utility and grocery bills, U.S. residents are experiencing the economic impacts of the crisis, while at the same time assessing and responding to health risks. The project team has a unique opportunity to study these shifting household consumption and behavioral responses and quantify the associated economic and environmental impacts. The team will collect household food, energy, and water consumption data as well as survey response data from 180 participating households in one Midwestern county and compare it to data collected before the stay-at-home orders were put in place.