This is part of a series of short introductions about College students, faculty, and staff that we would like to include in the Weekly Download. Would you like to be featured? Send a photo and some background info about yourself to computing@mtu.edu.
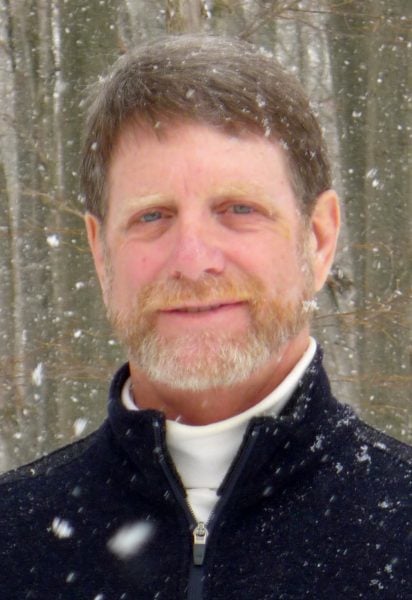
Dr. Robert Pastel, Associate Professor of Computer Science
- Advisor to Humane Interface Design Enterprise (HIDE)
- Has been teaching at Michigan Tech for about 20 years, and teaching for 30 years.
- Researcher with the Human-Centered Computing group of the Institute of Computing and Cybersystems (ICC)
Education
- PhD, University of New Mexico, Physics
- MS, Computer Science, Michigan Tech
Classes Dr. Pastel teaches:
o CS5760 – Human-Computer Interaction – Usability Evaluation and Testing
o CS4791 and CS4792 – Senior Design
o ENT1960 – ENT5960 – Humane Interface Design Enterprise
The “coolest” class you teach, and why: All my classes are “cool” because they all involve making applications that will be used by people. The “coolest” class is CS4760 – User Interface – Design and Implementation where students work with scientists across the world to make citizen science applications.
The importance of your class topics to the overall understanding of Computing and your discipline: In all my classes, students learn to design and implement usable applications for people.
Your teaching philosophy: My teaching philosophy is that students learn best by experience and working with others. Consequently students work in teams on project for clients.
Research projects in which students are assisting:
- StreamCLIMES – Large collaborative project studying bio diversity of intermittent streams. I’m responsible for developing a web applications monitoring the stream.
- FloodAware – Large collaborative project recording and modelling flooding in urban areas. I’m responsible for developing the citizen science effort.
- KeTT – Keweenaw Time Traveler – Historical geospatial information citizen science website for user to record region’s history and explore the maps and stories.
Interests beyond teaching and research: The outdoors: skiing, biking and hiking. Every summer, he takes a one-month backpacking trip.