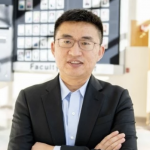
Associate Professor Kuilin Zhang, Civil and Environmental Engineering and affiliated associate professor, Computer Science, was featured in a recent article on Michigan Tech News. The article appears below. Link to the original article here.
By Kelley Christensen, September 28, 2020.
Networked data-driven vehicles can adapt to road hazards at longer range, increasing safety and preventing slowdowns.
Vehicle manufacturers offer smart features such as lane and braking assist to aid drivers in hazardous situations when human reflexes may not be fast enough. But most options only provide immediate benefits to a single vehicle. What if entire groups of vehicles could respond? What if instead of responding solely to the vehicle immediately in front of us, our cars reacted proactively to events happening hundreds of meters ahead?
What if, like a murmuration of starlings, our cars and trucks moved cooperatively on the road in response to each vehicle’s environmental sensors, reacting as a group to lessen traffic jams and protect the humans inside?
This question forms the basis of Kuilin Zhang’s National Science Foundation CAREER Award research. Zhang, an associate professor of civil and environmental engineering at Michigan Technological University, has published “A distributionally robust stochastic optimization-based model predictive control with distributionally robust chance constraints for cooperative adaptive cruise control under uncertain traffic conditions” in the journal Transportation Research Part B: Methodological.
The paper is coauthored with Shuaidong Zhao ’19, now a senior quantitative analyst at National Grid, where he continues to conduct research on the interdependency between smart grid and electric vehicle transportation systems.
Vehicle Platoons Operate in Sync
Creating vehicle systems adept at avoiding traffic accidents is an exercise in proving Newton’s First Law: An object in motion remains so unless acted on by an external force. Without much warning of what’s ahead, car accidents are more likely because drivers don’t have enough time to react. So what stops the car? A collision with another car or obstacle — causing injuries, damage and in the worst case, fatalities.
But cars communicating vehicle-to-vehicle can calculate possible obstacles in the road at increasing distances — and their synchronous reactions can prevent traffic jams and car accidents.
“On the freeway, one bad decision propagates other bad decisions. If we can consider what’s happening 300 meters in front of us, it can really improve road safety. It reduces congestion and accidents.”Kuilin Zhang
Zhang’s research asks how vehicles connect to other vehicles, how those vehicles make decisions together based on data from the driving environment and how to integrate disparate observations into a network.
Zhang and Zhao created a data-driven, optimization-based control model for a “platoon” of automated vehicles driving cooperatively under uncertain traffic conditions. Their model, based on the concept of forecasting the forecasts of others, uses streaming data from the modeled vehicles to predict the driving states (accelerating, decelerating or stopped) of preceding platoon vehicles. The predictions are integrated into real-time, machine-learning controllers that provide onboard sensed data. For these automated vehicles, data from controllers across the platoon become resources for cooperative decision-making.
CAREER Award
Kuilin Zhang won an NSF CAREER Award in 2019 for research on connected, autonomous vehicles and predictive modeling.
Proving-Grounds Ready
The next phase of Zhang’s CAREER Award-supported research is to test the model’s simulations using actual connected, autonomous vehicles. Among the locations well-suited to this kind of testing is Michigan Tech’s Keweenaw Research Center, a proving ground for autonomous vehicles, with expertise in unpredictable environments.
Ground truthing the model will enable data-driven, predictive controllers to consider all kinds of hazards vehicles might encounter while driving and create a safer, more certain future for everyone sharing the road.
Tomorrow Needs Mobility
Michigan Technological University is a public research university, home to more than 7,000 students from 54 countries. Founded in 1885, the University offers more than 120 undergraduate and graduate degree programs in science and technology, engineering, forestry, business and economics, health professions, humanities, mathematics, and social sciences. Our campus in Michigan’s Upper Peninsula overlooks the Keweenaw Waterway and is just a few miles from Lake Superior.
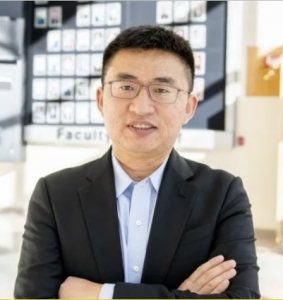
About the Researcher: Kuilin Zhang
- Data-driven optimization and control models for connected and automated vehicles (CAVs)
- Big traffic data analytics using machine learning
- Mobile and crowd sensing of dynamic traffic systems
- Dynamic network equilibrium and optimization
- Modeling and simulation of large-scale complex systems
- Freight logistics and supply chain systems
- Impact of plug-in electric vehicles to smart grid and transportation network systems
- Interdependency and resiliency of large-scale networked infrastructure systems
- Vehicular Ad-hoc Networks (VANETs)
- Smart Cities
- Cyber-Physical Systems