Timothy Havens (ECE/ICC) and Anthony Pinar (ECE) coauthored the article, “Enabling Explainable Fusion in Deep Learning with Fuzzy Integral Neural Networks,” which was accepted this month for publication in the journal IEEE Transactions on Fuzzy Systems.
DOI: 10.1109/TFUZZ.2019.2917124
Extract
Information fusion is an essential part of numerous engineering systems and biological functions, e.g., human cognition.
Fusion occurs at many levels, ranging from the low-level combination of signals to the high-level aggregation of heterogeneous decision-making processes.
While the last decade has witnessed an explosion of research in deep learning, fusion in neural networks has not observed the same revolution.
Herein, we prove that the fuzzy Choquet integral (ChI), a powerful nonlinear aggregation function, can be represented as a multi-layer network, referred to hereafter as ChIMP. An additional benefit of ChIMP/iChIMP is that it enables eXplainable AI (XAI).
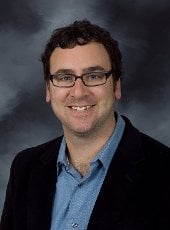
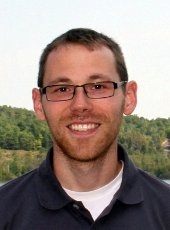