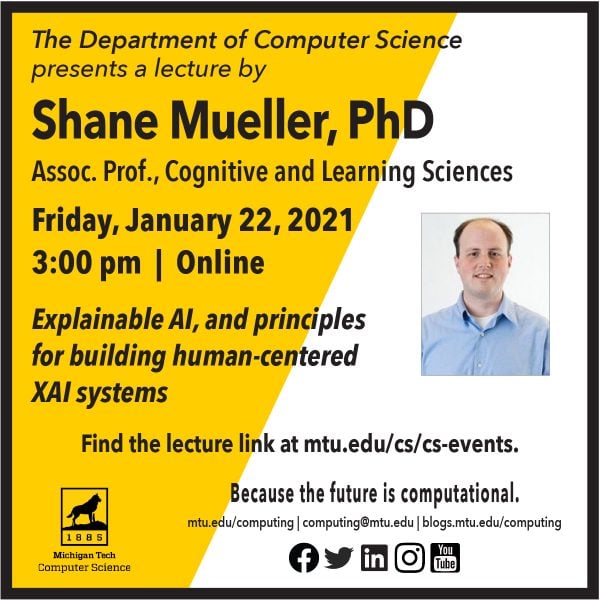
The Department of Computer Science will present a lecture, by Dr. Shane Mueller on Friday, January 22, 2021, at 3:00 p.m.
Mueller is an associate professor in the Applied Cognitive Science and Human Factors program of the Cognitive and Learning Science department. His lecture is titled, “Explainable AI, and principles for building human-centered XAI systems.”
Mueller’s research focuses on human memory and the representational, perceptual, strategic, and decisional factors that support it. He employs applied and basic research methodologies, typically with a goal of implementing formal quantitative mathematical or computational models of cognition and behavior.
He is also the primary developer of the Psychology Experiment Building Language (PEBL), a software platform for creating psychology experiments.
Mueller has undergraduate degrees in mathematics and psychology from Drew University, and a Ph.D. in cognitive psychology from the University of Michigan. He was a senior scientist at Klein Associates Division of Applied Research Associates from 2006 to 2011. His research has been supported by NIH, DARPA, IARPA, the Air Force Research Laboratory, the Army Research Institute, the Defense Threat Reduction Agency, and others.
Lecture Title:
Explainable AI, and principles for building human-centered XAI systems
Lecture Abstract
In recent years, Explainable Artificial Intelligence (XAI) has re-emerged in response to the development of modern AI and ML systems. These systems are complex and sometimes biased, but they nevertheless make decisions that impact our lives. XAI systems are frequently algorithm-focused; starting and ending with an algorithm that implements a basic untested idea about explainability. These systems are often not tested to determine whether the algorithm helps users accomplish any goals, and so their explainability remains unproven. I will discuss some recent advances and approaches to developing XAI, and describe how many of these systems are likely to incorporate many of the lessons from past successes and failures to build explainable systems. I will then review some of the basic concepts that have been used for user-centered XAI systems over the past 40 years of research. Based on this, I will describe a set of empirically-grounded, human user-centered design principles that may guide developers to create successful explainable systems.