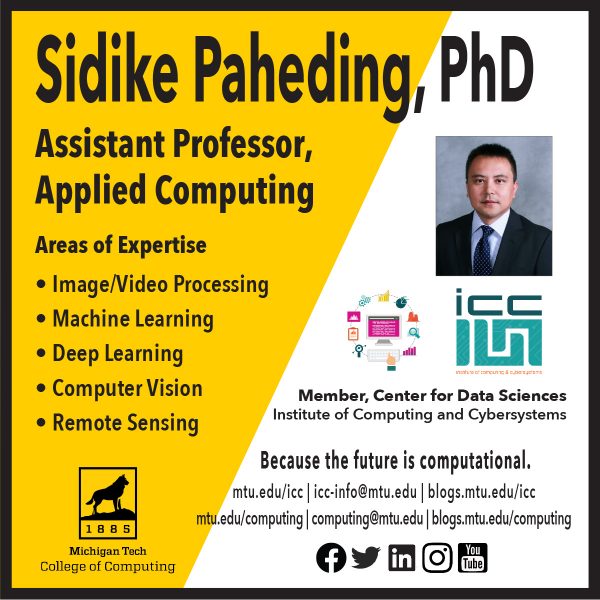
Sidike Paheding (AC/ICC) is the principal investigator on a project that has received a $19,037 research and development grant from Purdue University. The two-year project is titled, “Cybersecurity Modules Aligned with Undergraduate Computer Science and Engineering Curricula.”
The project aims to serve the national interest by improving how cybersecurity concepts are taught in undergraduate computing curricula.
The grant is a sub-award of a $159,417 Purdue University NSF project . View that project here.
Abstract
This project aims to serve the national interest by improving how cybersecurity concepts are taught in undergraduate computing curricula. The need to design and maintain cyber-secure computing systems is increasingly important. As a result, the future technology workforce must be trained to have a security mindset, so that they consider cybersecurity during rather than after system design. This project aims to achieve this goal by building plug-and-play, hands-on cybersecurity modules for core courses in Computer Engineering, and Computer Science and Engineering. The modules will align with the curricula recommended by the Association for Computing Machinery and will be designed for easy adoption into computing programs nationwide. Modules will be designed for integration into both introductory and advanced courses, thus helping students develop in-depth understanding of cybersecurity as they progress through their computing curriculum. It is expected that the project will encourage more students to pursue careers or higher degrees in the field of cybersecurity.
The project will examine how the modules may be best integrated into existing curricula and the effects of the modules on student learning and interest in cybersecurity. Assessment will leverage several methods including (a) a task load index to quantify rigor, (b) surveys to gain insight into the development of students’ security mindset and perceptions of cybersecurity, and (c) analysis of learning using analytical course rubrics. Deliverables of this project will include a suite of plug-and-play cybersecurity modules for Computer Engineering and Computer Science and Engineering courses that span from introductory to advanced levels and that meet standards for content breadth and depth. The results will be disseminated through publications, presentations, press releases, and social media to ensure that project outcomes are shared widely. The NSF Improving Undergraduate STEM Education: Education and Human Resources Program supports research and development projects to improve the effectiveness of STEM education for all students. Through the Engaged Student Learning track, the program supports the creation, exploration, and implementation of promising practices and tools.
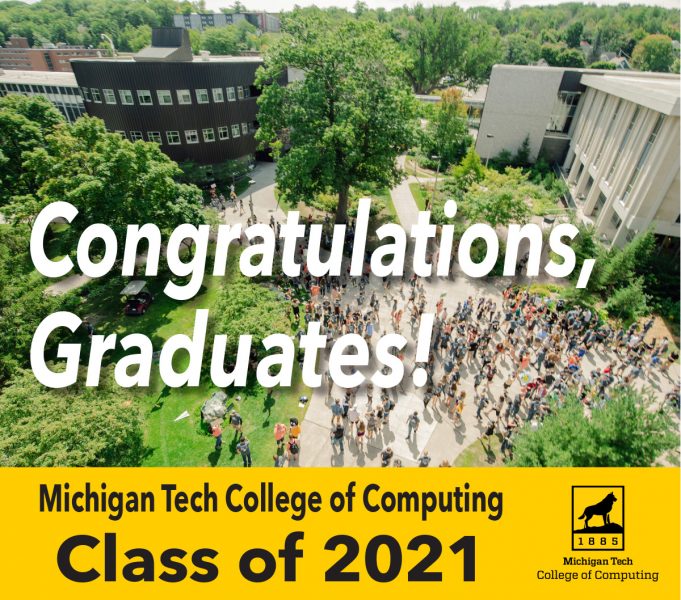
It has been a challenging academic year, to say the least. As part of the Class of 2021, you are an exceptional group of graduates. Your final academic year presented you with unforeseen and unprecedented challenges, yet you persevered.
We are all proud to have mentored, instructed, and supported you on your educational journey. We know you’ll do well. You are a Husky, after all!
Please stay in touch!
Nathir Rawashdeh (AC) led the publication of a paper at the recent online SPIE Defense + Commercial Sensing / Autonomous Systems 2021 Conference.
The paper, entitled “Drivable path detection using CNN sensor fusion for autonomous driving in the snow,” targets the problem of drivable path detection in poor weather conditions including on snow-covered roads. The authors used artificial intelligence to perform camera, radar and LiDAR sensor fusion to detect a drivable path for a passenger car on snow-covered streets. A companion video is available.
Co-authors include Jeremy Bos (ECE).
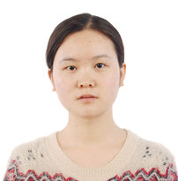
Jingjing Yao, a PhD candidate at New Jersey Institute of Technology, will present a talk on Tuesday, May 11, at 3:00 p.m,.
In her talk, “Intelligent and Secure Fog-Aided Internet of Drones,” Yao discusses the utilization of energy harvesting technology to charge drone batteries and investigate wireless power control to adjust the drone wireless transmission power to reduce drone energy consumption.
Yao’s research interests include Internet of Things (IoT), Internet of Drones (IoD), Deep Reinforcement Learning, Federated Learning, Cybersecurity, Mobile Edge Computing/Caching, and Energy Harvesting.
Talk Title
Intelligent and Secure Fog-Aided Internet of Drones
Talk Abstract
Internet of drones (IoD), which deploys several drones in the air to collect ground information and send them to the IoD gateway for further processing, can be applied in traffic surveillance and disaster rescue. Fog-aided IoD provisions future events prediction and image classification by machine learning technologies, where massive training data are collected by drones and analyzed in the fog node. However, the performance of IoD is greatly affected by drones’ battery capacities. Also, aggregating all data in the fog node may incur huge network traffic and drone data privacy leakage. The speaker will share her vision and research to address these two challenges.
In this talk, the speaker utilizes energy harvesting technology to charge drone batteries and investigate wireless power control to adjust the drone wireless transmission power to reduce drone energy consumption. The joint optimization of power control and energy harvesting scheduling is investigated in time-varying IoD networks to minimize the long-term average system energy cost constrained by the drone battery capacities and quality of service (QoS) requirements. A modified actor-critic deep reinforcement learning algorithm is designed to address the joint optimization problem in time-varying IoD networks.
To prevent the privacy leakage of IoD, the speaker utilizes federated learning (FL) by performing local training in drones and sharing all training model parameters in the fog node without uploading drone raw data. However, drone privacy can still be divulged to ground eavesdroppers by wiretapping and analyzing uploaded parameters during the FL training process. The power control problem is hence investigated to maximize the FL system security rate constrained by drone battery capacities and the FL training time requirement. An algorithm with low computational complexity is then designed to tackle the security rate maximization problem and its performance is demonstrated by extensive simulations.
Biography
Jingjing Yao is a Ph.D. candidate in Computer Engineering with the Department of Electrical and Computer Engineering at the New Jersey Institute of Technology (NJIT). She will receive her Ph.D. degree from NJIT in May 2021.
She received the M.E. degree in Information and Communication Engineering from the University of Science and Technology of China (USTC), and the B.E. degree in Information and Communication Engineering from the Dalian University of Technology (DUT).
She has published 13 first-author journal articles and 7 first-author conference papers. Her research interests include Internet of Things (IoT), Internet of Drones (IoD), Deep Reinforcement Learning, Federated Learning, Cybersecurity, Mobile Edge Computing/Caching, and Energy Harvesting.
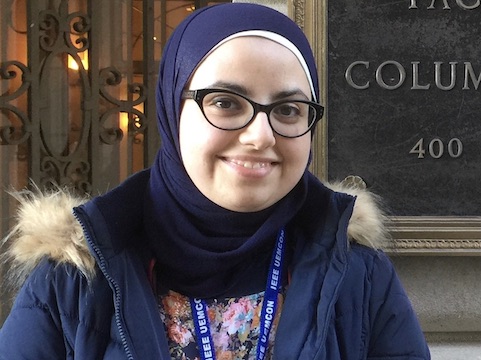
Tara Salman, a final-year PhD candidate at Washington University in St. Louis, will present a talk on Tuesday, April 27, 2021, at 3:00 p.m.
In her talk, “A Collaborative Knowledge-Based Security Solution using Blockchains,” she will present her work on redesigning the blockchains and building a collaborative, distributed, intelligent, and hostile solution that can be used for security purposes.
Talk Title
A Collaborative Knowledge-Based Security Solution using Blockchains
Talk Abstract
Artificial intelligence and machine learning have recently gained wide adaptation in building intelligent yet simple and proactive security solutions such as intrusion identification, malware detection, and threat intelligence. With the increased risk and severity of cyber-attacks and the distributed nature of modern threats and vulnerabilities, it becomes critical to pose a distributed intelligent solution that evaluates the systems’ and networks’ security collaboratively. Blockchain, as a decade-old successful distributed ledger technology, has the potential to build such collaborative solutions. However, to be used for such solutions, the technology needs to be extended so that it can intelligently process the stored information and achieve a collective decision about security risks or threats that might target a system.
In this talk, I will present our work on redesigning the blockchains and build a collaborative, distributed, intelligent, and hostile solution that can be used for security purposes. In particular, we will discuss our work on (1) extending blockchains for general collaborative decision-making applications, where knowledge should be made out of decisions, risks, or any information stored on the blockchain; (2) applying the proposed extensions to security applications such as malware detection and threat intelligence.
Biography
Tara Salman is a final year Ph.D. candidate at Washington University in St. Louis, where she is advised by Raj Jain. She previously received her MS and BSc degrees from Qatar University in 2015 and 2012, respectively. Her research aims to integrate state-of-the-art technologies to provide scalable, collaborative, and intelligent cybersecurity solutions.
Her recent work focuses on the intersection of artificial intelligence, blockchains, and security applications. The work spans several fields, including blockchain technology, security, machine learning, and deep learning applications, cloud computing, and the Internet of Things. She has been selected for the EECS Rising Star in UC Berkeley 2020. Her research has been published in more than twenty internationally recognized conferences and journals and supported by national and international funds.
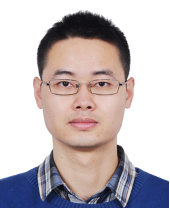
Xinyu Lei, a Ph.D. candidate in the Department of Computer Science and Engineering at Michigan State University, will present a talk on Thursday, April 29, 2021, at 3:00 p.m.
In his talk, “Secure and Efficient Queries Processing in Cloud Computing,” Lei will discuss his work developing new techniques to support secure and efficient queries processing in cloud storage.
Title
Secure and Efficient Queries Processing in Cloud Computing
Abstract
With the advent of cloud computing, data owners are motivated to outsource their databases to the commercial public cloud for storage. The public cloud with database-as-a-service (DBaaS) model has many benefits (including lower cost, better performance, and higher flexibility). However, hosting the datasets on the commercial public cloud deprives the data owners’ direct control over their databases, which brings in security concerns. For example, the corrupted cloud employees may spy the data owner’s commercial valuable databases and sell them for money. To protect data privacy, the sensitive database must be encrypted before outsourcing to the cloud. However, it becomes hard to perform efficient queries (e.g., keyword query) processing over the encrypted database.
In this talk, I focus on developing new techniques to support secure and efficient queries processing in cloud storage. An index-aid approach is proposed to address the problem. In my approach, the data items are formally encrypted, and a secure index is generated for efficient queries processing. The cloud can perform queries directly over the secure index rather than the encrypted data items. The secure index is constructed based on a new data structure named random Bloom filter. Then, multiple random Bloom filters are organized into a binary tree structure to support fast query processing. The proposed approach can achieve data privacy, index privacy, search token privacy, and fast query processing simultaneously.
Biography
Xinyu Lei is a Ph.D. candidate in the Department of Computer science and Engineering at Michigan State University. He once worked as a research assistant in Texas A&M University at Qatar and Ford Motor Company. He has research interests in cybersecurity problems in different computer systems (including IoT, blockchain, cloud computing). He has published 20+ papers with 600+ citations. His work has been published on top-tier conferences and journals such as ACM MobiSys, ACM CodaSpy, IEEE ICDE, IEEE ICDCS, etc.
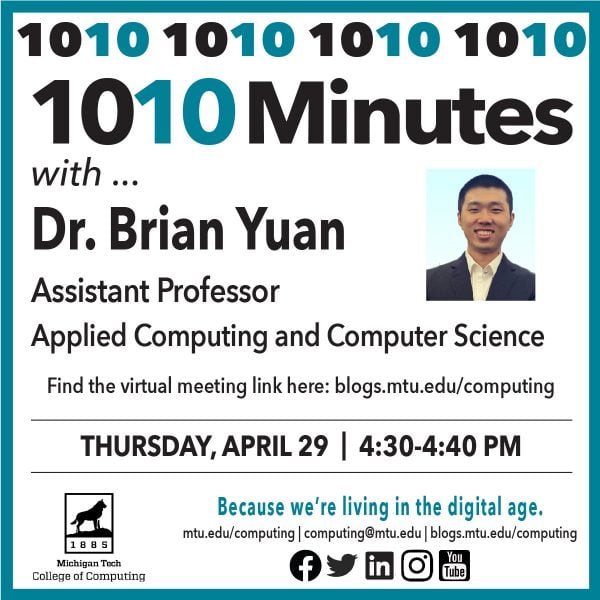
You are invited to spend one-zero-one-zero—that is, ten—minutes with Dr. Brian Yuan on Thursday, April 29, at 4:30 p.m. EST.
Dr. Yuan is an assistant professor in both the Applied Computing and Computer Science departments. His areas of expertise include machine learning, security and privacy, and cloud computing.
Yuan will discuss his research, the Applied Computing and Computer Science departments, and answer questions.
Dr. Yuan earned his PhD in Computer Science at University of Florida.
We look forward to spending 1010 minutes with you!