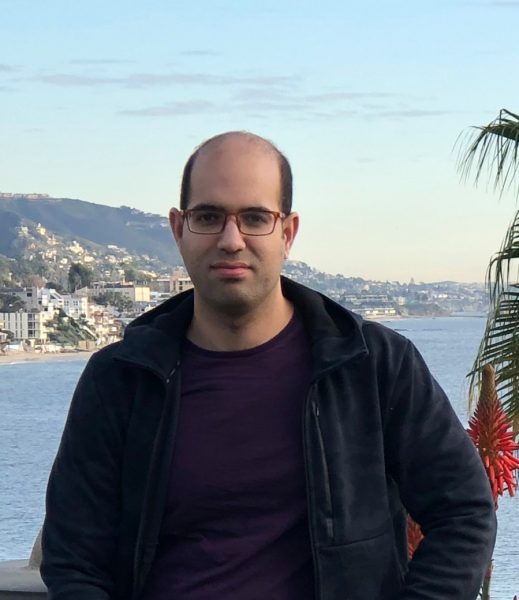
Dr. Ali Yekkehkhany, a postdoctoral scholar at the University of California, Berkeley, will present a talk on Thursday, May 6, 2021, at 3:00 p.m.
He will discuss adversarial attacks on the computation of reinforcement learning and risk-aversion in games and online learning.
Dr. Yekkehkhany’s research interests include machine/reinforcement learning, queueing theory, applied probability theory and stochastic processes.
Talk Title
Adversarial Reinforcement Learning, Risk-Averse Game Theory and Online Learning with Applications to Autonomous Vehicles and Financial Investments
Talk Abstract
In this talk, we discuss:
- a) Adversarial attacks on the computation of reinforcement learning: The emergence of cloud, edge, and fog computing has incentivized agents to offload the large-scale computation of reinforcement learning models to distributed servers, giving rise to edge reinforcement learning (RL). By the inherently distributed nature of edge RL, the swift shift to this technology brings a host of new adversarial attack challenges that can be catastrophic in safety-critical applications. A natural malevolent attack could be to contaminate the RL computation such that the contraction property of the Bellman operator is undermined in the value/policy iteration methods. This can result in luring the agent to search among suboptimal policies without improving the true values of policies. We prove that under certain conditions, the attacked value/policy iteration methods converge to the vicinity of the optimal policy with high probability if the number of value/policy evaluation iterations is larger than a threshold that is logarithmic in the inverse of a desired precision.
- b) Risk-aversion in games and online learning: The fast-growing market of autonomous vehicles, unmanned aerial vehicles, and fleets in general necessitates the design of smart and automatic navigation systems considering the stochastic latency along different paths in a traffic network. To our knowledge, the existing navigation systems including Google Maps, Waze, MapQuest, Scout GPS, Apple Maps, and others are based on minimizing the expected travel time, ignoring the path delay uncertainty. To put the travel time uncertainty into perspective, we model the decision making of risk-averse travelers in a traffic network by an atomic stochastic congestion game and propose three classes of risk-averse equilibria. We show that the Braess paradox may not occur to the extent presented originally and the price of anarchy can be improved, benefiting the society, when players travel according to risk-averse equilibria rather than the Wardrop/Nash equilibrium. Furthermore, we extend the idea of risk-aversion to online learning; in particular, risk-averse explore-then-commit multi-armed-bandits. We use data from the New York Stock Exchange (NYSE) to show that the classical mean-variance and conditional value at risk approaches can come short in addressing risk-aversion for financial investments. We introduce new venues to study risk-aversion by taking the probability distributions into account rather than the summarized statistics of distributions.
Biography
Ali Yekkehkhany is a postdoctoral scholar with the Department of Industrial Engineering and Operations Research, University of California, Berkeley. He received his PhD and MSc degrees in Electrical and Computer Engineering from the University of Illinois, Urbana-Champaign (UIUC) in 2020 and 2017, respectively, and BSc degree in Electrical Engineering from Sharif University of Technology in 2014.
He is the recipient of the “best poster award in recognition of high-quality research, professional poster, and outstanding presentation” in the 15th CSL Student Conference, 2020, and the “Harold L. Olesen award for excellence in undergraduate teaching by graduate students” in the 2019-2020 academic year at UIUC. He was chosen as “teachers ranked as excellent” twice and “teachers ranked as excellent and outstanding” twice at UIUC.
His research interests include machine/reinforcement learning, queueing theory, applied probability theory and stochastic processes.