by Karen S. Johnson, Communications Director, College of Computing
The Challenge
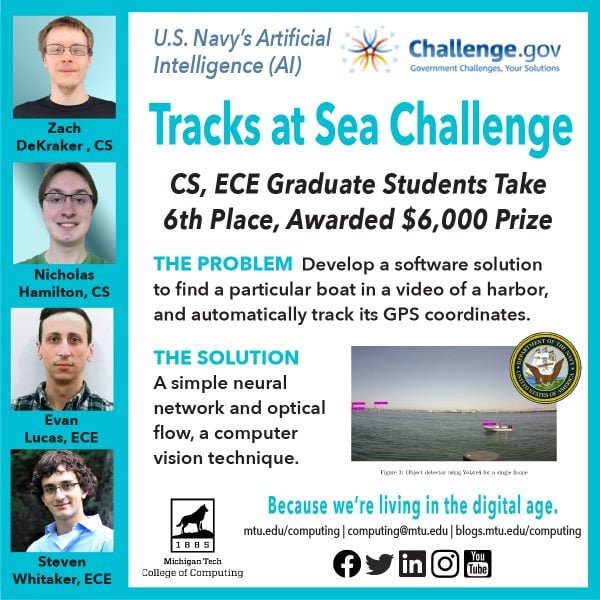
Four Michigan Tech graduate students recently took 6th place in the U.S. Navy’s Artificial Intelligence (AI) Tracks at Sea Challenge, receiving a $6,000 prize.
The Challenge solicited software solutions to automatically generate georeferenced tracks of maritime vessel traffic based on data recorded from a single electro-optical camera imaging the traffic from a moving platform.
Each Challenge team was presented with a dataset of recorded camera imagery of vessel traffic, along with the recorded GPS track of a vessel of interest that is seen in the imagery.
Graduate students involved in the challenge were Zach DeKraker and Nicholas Hamilton, both Computer Science majors advised by Tim Havens; Evan Lucas, Electrical Engineering, advised by Zhaohui Wang; and Steven Whitaker, Electrical Engineering.
Submitted solutions were evaluated against additional camera data not included in the competition testing set in order to verify generalization of the solutions. Judging was based on track accuracy (70%) and overall processing time (30%).
“We never got our final score, but we were the “first runner up” team,” says Lucas. “Based on our testing before sending it, we think it worked well most of the time and occasionally tracked a seagull or the wrong boat.”
The total $200,000 prize was distributed among five winning teams, which submitted full working solutions, and three runners-up, which submitted partial working solutions.
The Challenge was sponsored by the Naval Information Warfare Center (NIWC) Pacific and the Naval Science, Technology, Engineering, and Mathematics (STEM) Coordination Office, and managed by the Office of Naval Research. Its goal was to engage with the workforce of tomorrow on challenging and relevant naval problems, with the immediate need to augment unmanned surface vehicles’ (USVs’) maritime contact tracking capability.
The Problem
“The problem presented was to find a particular boat in a video taken of a harbor, and track its GPS coordinates.,” says Zach DeKraker. “We were provided with samples of other videos along with the target boat’s GPS coordinates for that video, which we were able to use to come up with a mapping from pixels to GPS coordinates.”
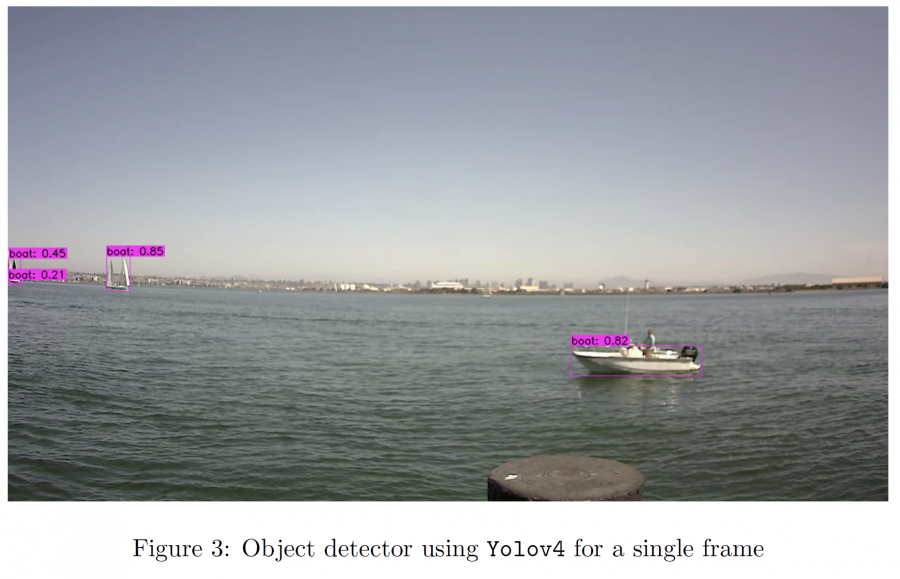
“Basically, we wanted to track boats with a video camera,” adds ECE graduate student Steven Whitaker. “Our team used machine learning and computer vision to do this. At weekly meetings we brainstormed approaches to tackling the problem, and at regular work sessions, together we programmed it all and produced a white paper with the technical details.”
Whitaker says the competition tied in pretty closely to work the students have already done. “We had a good majority of the code already written. We just needed to fit everything together and add in a few more details and specialize it for the AI Tracks at Sea research,” he explains.
Competitions like this one often connect directly or indirectly with a student’s academic and career goals.
“It’s good to not be pigeon-holed, and to use our knowledge in a different scenario,” Steven Whitaker says of these opportunities. “This helps us remember that there are other things in the world other than our small section of research.”
Dividing Responsibilities
The team knew that there were two primary issues at hand. First, how can the pixel coordinates be translated into GPS coordinates? And second, how can the boat be located so that GPS pixel coordinates can be determined?
“Once we broke it down into these two subproblems, it became pretty clear how to solve each half,” DeKraker says. “Steven had already done a significant amount of work mapping pixel coordinates into GPS coordinates, so we had a pretty quick answer to subproblem one.”
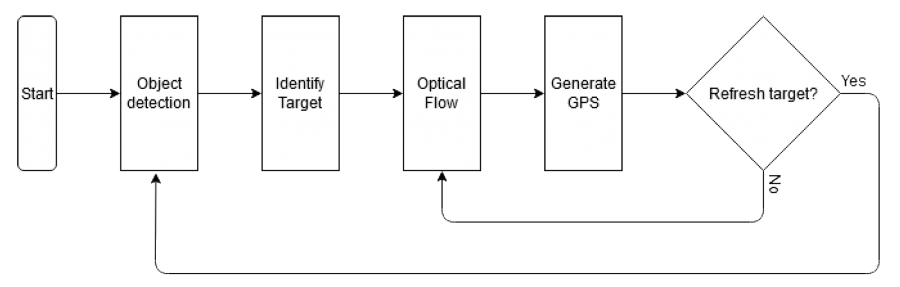
The team met weekly to discuss their ideas for the project and compare and contrast how effective they would be as solutions to the problem at hand. Then, they got together on Fridays or during the weekends to work together on the project.
“Dr. Havens would come in to our weekly meetings and nudge us in the right direction or give tips on what we should do and what we should avoid,” Whitaker adds.
For subproblem two, after some discussion the group decided it was probably best to use a machine learning approach, as that promised the most significant gains for the least amount of effort, which was important given the tight schedule.
“We tried some different sub-projects independently and then worked together to combine the parts we thought worked best,” Evan Lucas says.
The Solution
To identify the boat and track its movement, the team used a simple neural network and a computer vision technique called optical flow, which made the analysis much faster and cleaner. They used a pre-built algorithm, adding a bit of optical flow so that the boat’s position didn’t have to be verified every time.
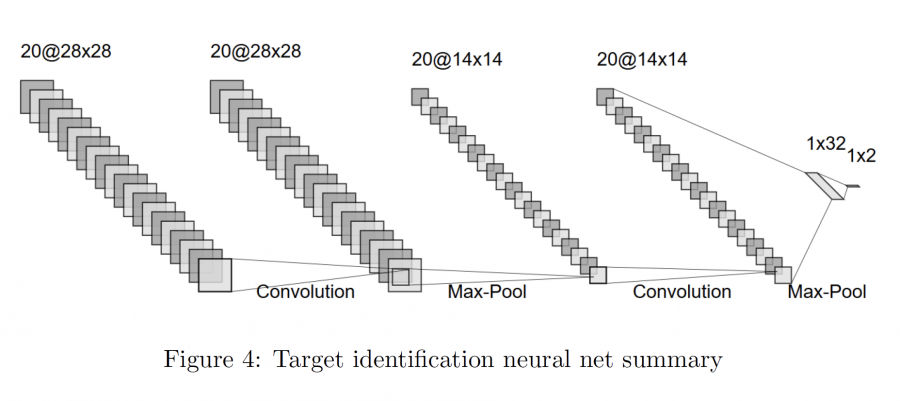
“These two tools allowed us to find the pixel coordinates of the boat and turn them into GPS coordinates,” DeKraker says, whose primary role in the project was integrating the two tools and packaging it for testing.
“Part of my PhD is to map out a snowmobile’s GPS coordinates with a camera,” Whitaker says. “This is extremely similar to mapping out a boat’s GPS coordinates. I could even say that it was exactly the same. I don’t believe I’ll add anything new, but I’ve tweaked it to work for my research.”
Whitaker sums up the team’s division of responsibilities like this: “Evan detects all the boats in the picture; Nik detects which of those boats is our boat; Steven takes our boat position and converts it to GPS coordinates, Zach glued all of our pieces together.”
DeKraker says, “One of the things the judges stressed was the ease of implementing the solution. Since that falls under what I would consider user experience (UX) or user interface (UI), it was pretty natural for me to take these tasks on, having studied software engineering for my undergrad,” DeKraker says.
A primary focus was speed. “Using machine learning for object detection tends to be slow, so to mitigate that we used the boat detector only once every 5 seconds,” DeKraker explains.
“Most of the tracking was done using a very fast technique called optical flow, which looks at the difference between two consecutive frames of a video to track motion,” DeKraker says. “It tended to drift from the target though, so we decided on running the boat detector every 5 seconds to keep optical flow on target. “
“The end result is that our solution could run nearly in real-time,” he says. “The accuracy wasn’t the best, but given a little bit more time and more training data, the neural network could be significantly improved.”
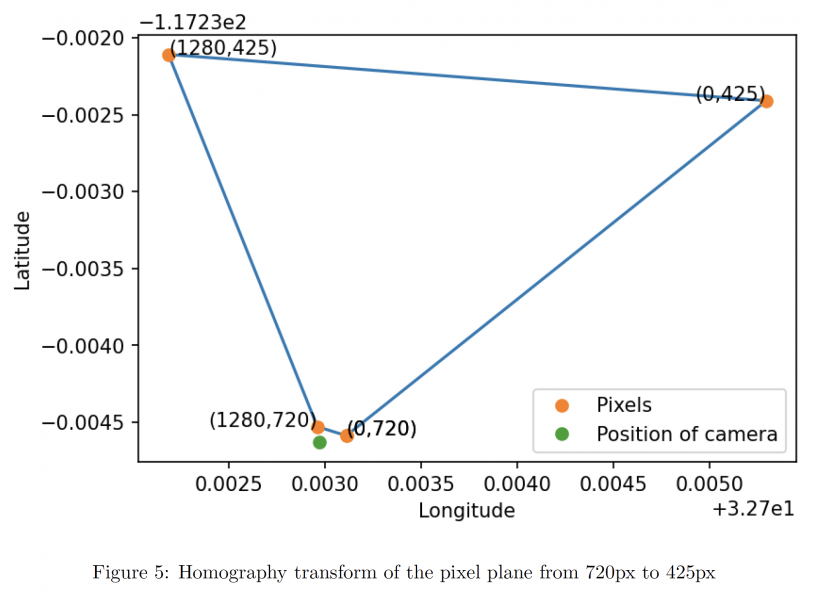
Zach DeKraker
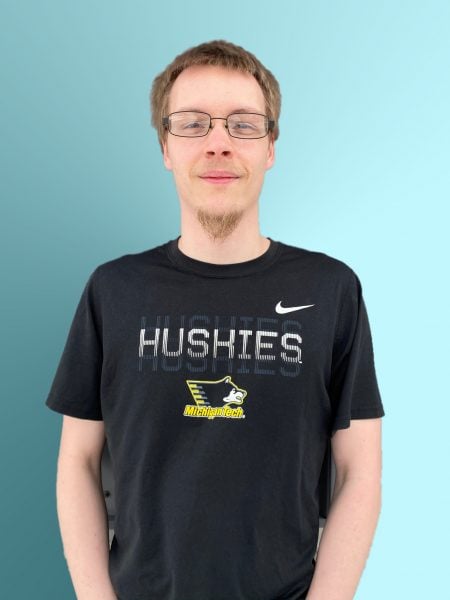
DeKraker’s graduate studies focus heavily on various machine learning techniques, He says that this opportunity to integrate machine learning into our solution was a fantastic experience.
“First, it sounded like an interesting challenge. I don’t get to do a lot of software design these days, and this challenge sounded like a great opportunity to do just that,” he explains.
“Second, it looked like a great opportunity to build up my resume a little bit. Saying that you won thousands of dollars for your university in a nationwide competition sounds really good. And finally, I really wanted the chance to see a practical application of machine learning in action.”
DeKraker completed a BS in Software Engineering at Michigan Tech in 2018. He returned to Michigan Tech the next year to complete his master’s degree. He says the biggest reason he did so was to learn more about machine learning.
“Before embarking on this journey, I really didn’t know anything about it,” he says of machine learning. “Having this chance to actually solve a problem, to integrate a neural network into a fully realized boat tracker using nothing but a video helped me see how machine learning can be used practically, rather than merely understanding how it works.”
And although it was a fascinating exploration into the practical side of machine learning and computer vision, DeKraker says it’s rather tangential to his main research focus right now, which is on comparing different network architectures to evaluate which one performs best given particular data and the problem being solved.
DeKraker believes that the culture is the most magnetizing thing about Tech. “Everybody here is cut from the same cloth. We’re all nerds and proud of it,” he explains. “You can have a half-hour conversation with a complete stranger about singularities, the economics of fielding a fleet of star destroyers, or how Sting was forged.”
And the most appealing thing about Michigan Tech was its size. DeKraker says. “When I looked at a ranking of the top universities in Michigan, Tech was number 3, but still extremely small. It was a perfect blend of being a small but very good school.”
And he says the second-best thing about Tech is the location. “The Keweenaw is one of the most beautiful places on earth.”
DeKraker has many ideas about where he’d like to take his career. For instance, he’d love the chance to work for DARPA, Los Alamos National Laboratory, or NASIC. He also intends to commission into the Air Force in the next couple of years, “if they have a place for programmers like me.”
Evan Lucas
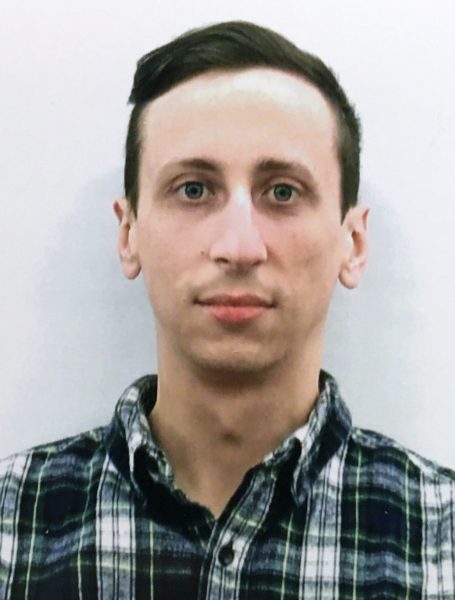
Evan Lucas is a PhD candidate in the Electrical Engineering department., advised by Zhaohui Wang. Lucas completed both a bachelor’s and master’s in Mechanical Engineering at Tech in 2012 and 2014,
Lucas, whose research interests are in applying machine learning methods to underwater acoustic communication systems, worked on developing a classifier to separate the boat of interest from the many other boats in the image. Although the subject of the competition is tangential to Lucas’s graduate studies, as computer vision isn’t his area, there was some overlap in general machine learning concepts. respectively.
“It sounded like a fun challenge to put together an entry and learn more about computer vision,” Lucas says. “Working with the rest of the team was a really good opportunity to learn from people who have experience making software that is used by other people.”
Following completion of his doctoral degree, hopefully in spring 2023, Lucas plans to return to industry in a research focused role that applies some of the work he did in his PhD.
Steven Whitaker
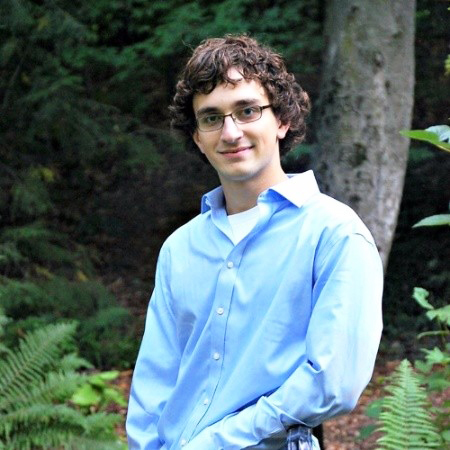
Steven Whitaker’s research interests are in machine learning and acoustics. He tracks and locates the position of on-ice vehicles, like snowmobiles, based on acoustics. He says he has used some of the results from this competition project in his PhD research.
Whitaker’s machine learning research is experiment-based., and that’s why he chose Michigan Tech. “There aren’t many opportunities in academia to do experiment-based research,” he says. “Most machine learning is very software-focused using pre-made datasets. I love doing the experiments myself. Research is fun. I enjoy getting paid to do what I normally would do in my free time.”
In 2019, Whitaker completed his BS in Electrical Engineering at Michigan Tech. He expects to complete his master’s degree in Electrical Engineering at the end of the summer 2021 semester, and his PhD in summer 2022. His advisors are Tim Havens and Andrew Barnard.
Whitaker would love to be a university professor one day, but first he wants to work in industry.
Background Info
Timothy Havens is associate dean for research, College of Computing; the William and Gloria Jackson Associate Professor of Computer Systems; and director of the Institute of Computing and Cybersystems (ICC). His research interests are in pattern recognition and machine learning, signal and image processing, sensor and data fusion, heterogeneous data mining, and explosive hazard detection.
Michael Roggeman is a professor in the Electrical and Computer Engineering department. His research interests include optics, image reconstruction and processing, pattern recognition, and adaptive and atmospheric optics.
Zhaohui Wang is an associate professor in the Electrical and Computer Engineering department. Her research interests are in communications, signal processing, communication networks, and network security, with an emphasis on underwater acoustic applications.
The Naval Information Warfare Center (NIWC) Pacific and the Naval Science, Technology, Engineering, and Mathematics (STEM) Coordination Office, managed by the Office of Naval Research are conducting the Artificial Intelligence (AI) Tracks at Sea challenge.
View more details about the Challenge competition here: https://www.challenge.gov/challenge/AI-tracks-at-sea/
Watch a Navy webinar about the Challenge here: https://www.youtube.com/watch?v=MjZwvCX4Tx0.
Challenge.gov is a web platform that assists federal agencies with inviting ideas and solutions directly from the public, or “crowd.” This is called crowdsourcing, and it’s a tenet of the Challenge.gov program. The website enables the U.S. government to engage citizen-solvers in prize competitions for top ideas and concepts as well as breakthrough software, scientific and technology solutions that help achieve their agency missions.
This site also provides a comprehensive toolkit, a robust repository of considerations, best practices, and case studies on running public-sector prize competitions as developed with insights from prize experts across government.
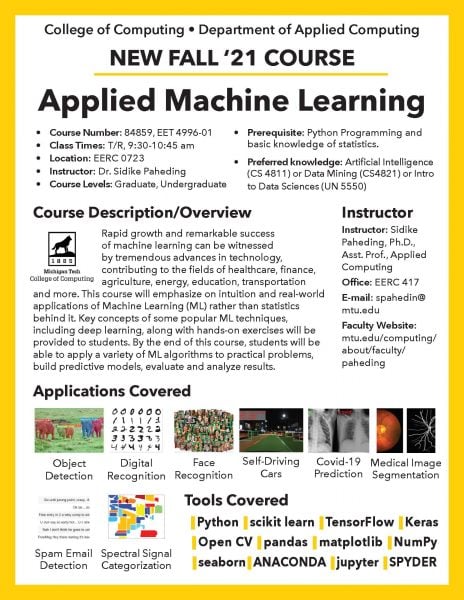
Summary
- Course Number: 84859, EET 4996-01
- Class Times: T/R, 9:30-10:45 am
- Location: EERC 0723
- Instructor: Dr. Sidike Paheding
- Course Levels: Graduate, Undergraduate
- Prerequisite: Python Programming and basic knowledge of statistics.
- Preferred knowledge: Artificial Intelligence (CS 4811) or Data Mining (CS4821) or Intro to Data Sciences (UN 5550)
Course Description/Overview
Rapid growth and remarkable success of machine learning can be witnessed by tremendous advances in technology, contributing to the fields of healthcare, finance, agriculture, energy, education, transportation and more. This course will emphasize on intuition and real-world applications of Machine Learning (ML) rather than statistics behind it. Key concepts of some popular ML techniques, including deep learning, along with hands-on exercises will be provided to students. By the end of this course, students will be able to apply a variety of ML algorithms to practical
Applications Covered
- Object Detection
- Digital Recognition
- Face Recognition
- Self-Driving Cars
- Medical Image Segmentation
- Covid-19 Prediction
- Spam Email Detection
- Spectral Signal Categorization
Tools Covered
- Python
- scikit learn
- TensorFlow
- Keras
- Open CV
- pandas
- matplotlib
- NumPy
- seaborn
- ANACONDA
- jupyter
- SPYDER
Download the course description flyer:
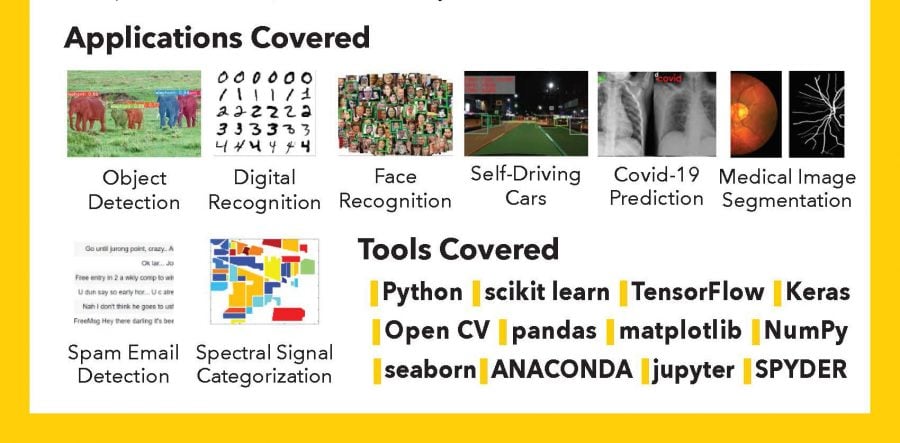
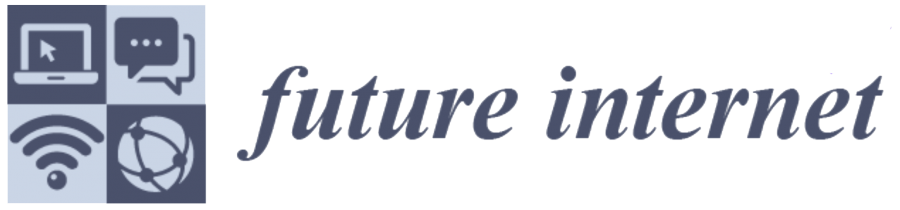
Call for Manuscripts:
Special Issue on Fault Tolerance in Cloud/Edge/Fog Computing in Future Internet, an international peer-reviewed open access monthly journal published by MDPI.
Informational Flyer
https://blogs.mtu.edu/icc/files/2021/04/ali-ebnenasir-call-for-papers-032521-sm.pdf
Deadline
April 20, 2021
Author Notification
June 10, 2021
Website
mdpi.com/journal/futureinternet/special_issues/FT_CEFC
Collection Editors
- Dr. Ali Ebnenasir, Michigan Technological University
- Dr. Sandeep S. Kulkarni, Michigan State University
Keywords
- Fault tolerance
- Cloud computing
- Edge computing
- Resource-constrained devices
- Distributed protocols
- State replication
Topics
Including, but not limited to:
- Faults and failures in cloud and edge computing.
- State replication on edge devices under the scarcity of resources.
- Fault tolerance mechanism on the edge and in the cloud.
- Models for the predication of service latency and costs in distributed fault-tolerant protocols on the edge and in the cloud.
- Fault-tolerant distributed protocols for resource management of edge devices.
- Fault-tolerant edge/cloud computing.
- Fault-tolerant computing on low-end devices.
- Load balancing (on the edge and in the cloud) in the presence of failures.
- Fault-tolerant data intensive applications on the edge and the cloud.
- Metrics and benchmarks for the evaluation of fault tolerance mechanisms in cloud/edge computing.
Background
The Internet of Things (IoT) has brought a new era of computing that permeates in almost every aspect of our lives. Low-end IoT devices (e.g., smart sensors) are almost everywhere, monitoring and controlling the private and public infrastructure (e.g., home appliances, urban transportation, water management system) of our modern life. Low-end IoT devices communicate enormous amount of data to the cloud computing centers through intermediate devices, a.k.a. edge devices, that benefit from stronger computational resources (e.g., memory, processing power).
To enhance the throughput and resiliency of such a three-tier architecture (i.e., low-end devices, edge devices and the cloud), it is desirable to perform some tasks (e.g., storing shared objects) on edge devices instead of delegating everything to the cloud. Moreover, any sort of failure in this three-tier architecture would undermine the quality of service and the reliability of services provided to the end users.
Scope
Theoretical and experimental methods that incorporate fault tolerance in cloud and edge computing, which have the potential to improve the overall robustness of services in three-tier architectures.
Manuscript Submission Information
Manuscripts should be submitted online at www.mdpi.com by registering and logging in to this website (https://www.mdpi.com/user/login/). Once you are registered, click here to go to the submission form (https://susy.mdpi.com/user/manuscripts/upload/?journal=futureinternet).
Manuscripts can be submitted until the deadline. All papers will be peer-reviewed. Accepted papers will be published continuously in the journal (as soon as accepted) and will be listed together on the special issue website. Research articles, review articles as well as short communications are invited. For planned papers, a title and short abstract (about 100 words) can be sent to the Editorial Office for announcement on this website.
Submitted manuscripts should not have been published previously, nor be under consideration for publication elsewhere (except conference proceedings papers). All manuscripts are thoroughly refereed through a single-blind peer-review process. A guide for authors and other relevant information for submission of manuscripts is available on the Instructions for Authors page.
Please visit the Instructions for Authors page before submitting a manuscript.
The Article Processing Charge (APC) for publication in this open access journal is 1400 CHF (Swiss Francs). Submitted papers should be well formatted and use good English.
Authors may use MDPI’s English editing service prior to publication or during author revisions.
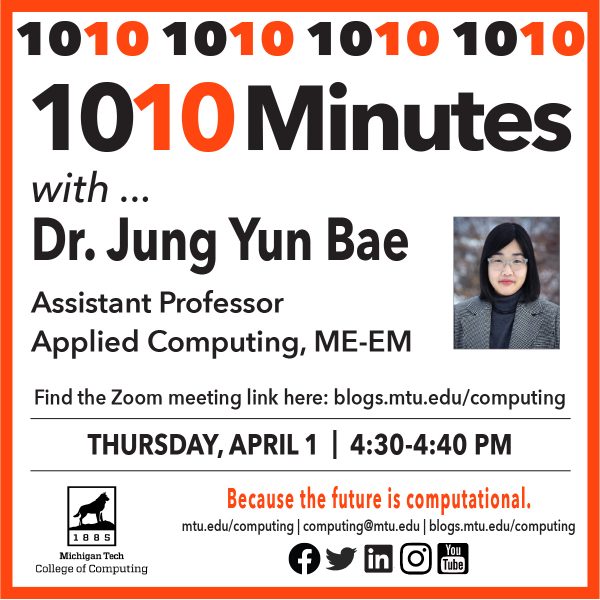
You are invited to spend one-zero-one-zero—that is, ten—minutes with Dr. Jung Yun Bae on Thursday, April 1, from 4:30 to 4:40 p.m. EST.
Dr. Bae is an Assistant Professor in the Applied Computing and Mechanical Engineering-Engineering Mechanics departments.
She will discuss her research, the Applied Computing department, and answer questions.
Dr. Bae earned her Ph.D. in Mechanical Engineering at Texas A&M University and worked as a research professor at Korea University before she joined Michigan Tech.
Dr. Bae’s research interests include:
- Robotics, Multi-robot systems
- Coordination of Heterogeneous Robot Systems
- Vehicle Routing Problems
- Multi-robot System Control and Optimization
- Autonomous Navigation
- Unmanned Vehicles
- Operational Research for Autonomous Vehicles
We look forward to spending 1010 minutes with you!
This is part of a series of short introductions about College students, faculty, and staff that we would like to include in the Weekly Download. Would you like to be featured? Send a photo and some background info about yourself to computing@mtu.edu.
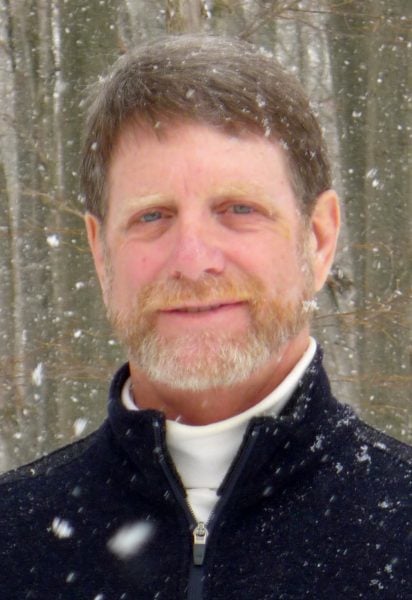
Dr. Robert Pastel, Associate Professor of Computer Science
- Advisor to Humane Interface Design Enterprise (HIDE)
- Has been teaching at Michigan Tech for about 20 years, and teaching for 30 years.
- Researcher with the Human-Centered Computing group of the Institute of Computing and Cybersystems (ICC)
Education
- PhD, University of New Mexico, Physics
- MS, Computer Science, Michigan Tech
Classes Dr. Pastel teaches:
o CS5760 – Human-Computer Interaction – Usability Evaluation and Testing
o CS4791 and CS4792 – Senior Design
o ENT1960 – ENT5960 – Humane Interface Design Enterprise
The “coolest” class you teach, and why: All my classes are “cool” because they all involve making applications that will be used by people. The “coolest” class is CS4760 – User Interface – Design and Implementation where students work with scientists across the world to make citizen science applications.
The importance of your class topics to the overall understanding of Computing and your discipline: In all my classes, students learn to design and implement usable applications for people.
Your teaching philosophy: My teaching philosophy is that students learn best by experience and working with others. Consequently students work in teams on project for clients.
Research projects in which students are assisting:
- StreamCLIMES – Large collaborative project studying bio diversity of intermittent streams. I’m responsible for developing a web applications monitoring the stream.
- FloodAware – Large collaborative project recording and modelling flooding in urban areas. I’m responsible for developing the citizen science effort.
- KeTT – Keweenaw Time Traveler – Historical geospatial information citizen science website for user to record region’s history and explore the maps and stories.
Interests beyond teaching and research: The outdoors: skiing, biking and hiking. Every summer, he takes a one-month backpacking trip.
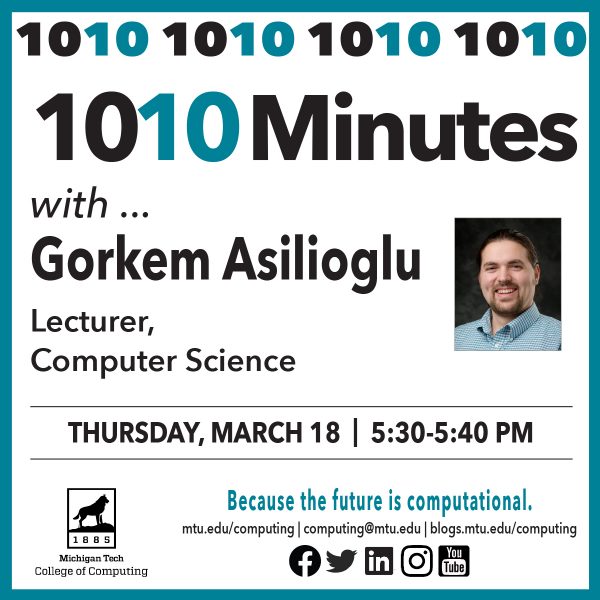
You are invited to spend one-zero-one-zero—that is, ten—minutes with Gorkem Asilioglu, Computer Science, on Thurday, March 18, from 5:30 to 5:40 p.m. EST.
Asilioglu is a lecturer in the Department of Computer Science. His research interests include computer architecture, high-performance computing, programming languages, and CS education.
We look forward to spending 1010 minutes with you!
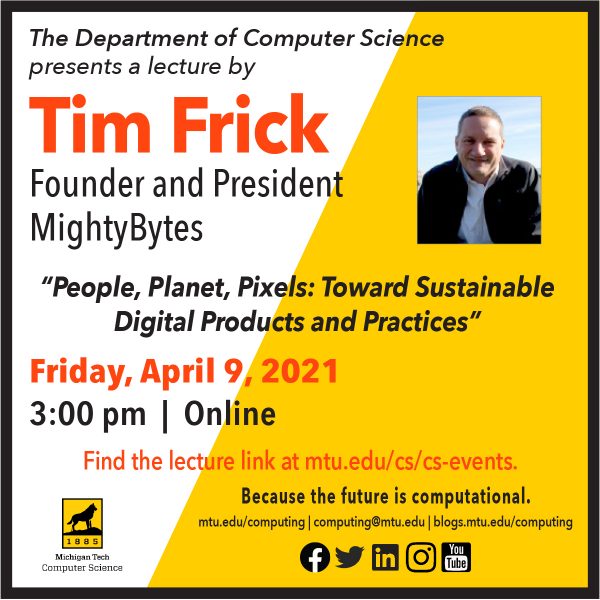
The Department of Computer Science will present a lecture by Tim Frick, founder and president of Mightybytes, on Friday, April 9, 2021, at 3:00 p.m.
In his talk, “People, Planet, Pixels: Toward Sustainable Digital Products and Practices,” Frick will discuss how sustainable web design and responsible digital practices can help create an internet that is clean, efficient, open, honest, regenerative, and resilient.
Lecture Title
“People, Planet, Pixels: Toward Sustainable Digital Products and Practices”
Speaker Bio
Tim Frick started his digital agency Mightybytes in 1998 to help purpose-driven companies, social enterprises, and large nonprofits solve problems, amplify their impact, and drive measurable results. He is the author of four books, including Designing for Sustainability: A Guide to Building Greener Digital Products and Services. Tim regularly presents at conferences and offers workshops on sustainable design, measuring impact, and problem solving in the digital economy.
Lecture Abstract
The internet has a larger environmental impact than the commercial airline industry. It currently produces approximately 3.8% of global carbon emissions, which are rising in line with our hunger to consume more data. Increasingly, web technologies are also being used to sow discontent, erode privacy, prompt unethical decisions, and, in some countries, undermine personal freedoms and the well-being of society. Web technology has the potential to bring huge benefits to society and the environment, but only if we use it wisely.
In this talk, author and digital agency owner Tim Frick will discuss how sustainable web designand responsible digital practices can help us create an internet that is clean, efficient, open, honest, regenerative, and resilient—principles outlined in the Sustainable Web Manifesto, of which Tim is a co-author. Elements of this talk are also based on Tim’s book, Designing for Sustainability: A Guide to Building Greener Digital Products and Services. Creating an internet that works for people and planet is possible. The methods described in this talk will show you how.
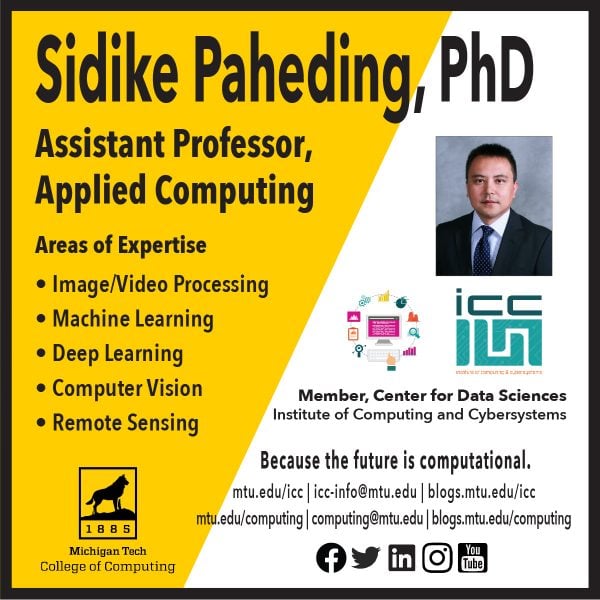
A scholarly paper co-authored by Assistant Professor Sidike Paheding, Applied Computing, has been published in the April 2021 issue of ISPRS Journal of Photogrammetry and Remote Sensing, published by Science Direct.
The title of the paper is, “Field-scale crop yield prediction using multi-temporal WorldView-3 and PlanetScope satellite data and deep learning.”
Paheding is a member of the Institute of Computer and Cybersystems’s (ICC) Center for Data Sciences.