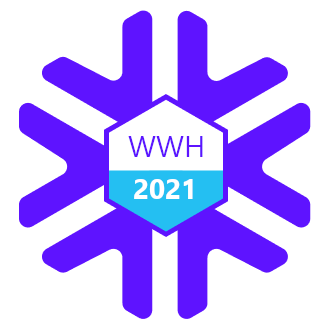
Winter WonderHack is just around the corner. The event begins February 19, 2021, at 9:00 p.m., and ends February 21, 2021, at 9:00 a.m.
Are you an up-and-coming coder? Do you have a coding project in mind you’ve been itching to finally bring to fruition? Or perhaps you have no ideas, but you need to add some new projects to your resume, something you can brag about to recruiters?
Do you like to win prizes? How about just hanging out and/or playing games?
Do all of the above and more at the virtual Winter WonderHack February 19-21.
The event is sponsored by the College of Computing, Wolfram Language, Auto Owners Insurance, and Domino’s Pizza.
“Okay, but what IS Winter WonderHack?” you may ask.
Winter WonderHack is a hackathon hosted annually by MTU’s very own Humane Interface Design Enterprise, or HIDE. It is an event that extends over 36 hours, in which groups ranging in size from one to four people come together to build and code any project from scratch–but it’s not all work and no play! There are a wide range of activities that will be occurring all day and all night for you to take a break from brainstorming and just have fun!
After the 36th hour on Sunday, all projects will be presented to a panel of judges, and then prizes will be awarded under a variety of categories, ranging from the Most Creative project to the Most Useless!
For the entire weekend, MTU’s Red Team will host a Capture the Flag event. On Saturday, a Rocket League tournament will occur! Both events will be conducted virtually via Discord. (For anyone worried about missing out, Rocket League is available free for download on PC, PS4, XBox One, and Nintendo Switch.)
All events during Winter Wonderhack are free, and all levels of technical backgrounds are welcome to attend! To register, visit the Winter Wonderhack website at https://winterwonderhack.com/#, and click on the Register link.
Do you have more questions about the event? Please reach out to team@winterwonderhack.com. We hope to see you there!