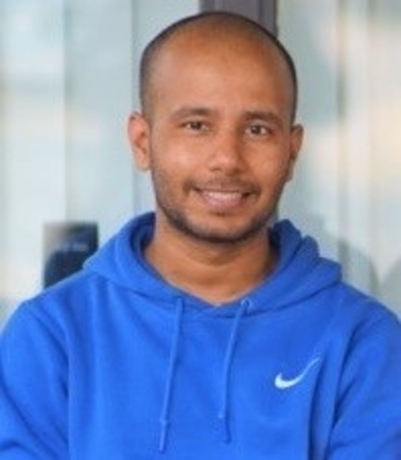
Graduate student Siva Krishna Kakula, Computer Science, will present his PhD defense, “Explainable Feature- and Decision-Level Fusion,” on Monday, December 21, 2020, from 3:00 to 5:00 p.m. EST Kakula is advised by Dr. Timothy Havens, College of Computing.
Siva Kakula earned his master of science in computer engineering at Michigan Tech in 2014, and completed a bachelor of technology in civil engineering at IIT Guwahati in 2011. His research interests include machine learning, pattern recognition, and information fusion.
Download the informational flier below.
Lecture Abstract
Information fusion is the process of aggregating knowledge from multiple data sources to produce more consistent, accurate, and useful information than any one individual source can provide. In general, there are three primary sources of data/information: humans, algorithms, and sensors. Typically, objective data—e.g., measurements—arise from sensors. Using these data sources, applications such as computer vision and remote sensing have long been applying fusion at different “levels” (signal, feature, decision, etc.). Furthermore, the daily advancement in engineering technologies like smart cars, which operate in complex and dynamic environments using multiple sensors, are raising both the demand for and complexity of fusion. There is a great need to discover new theories to combine and analyze heterogeneous data arising from one or more sources.
The work collected in this dissertation addresses the problem of feature- and decision-level fusion. Specifically, this work focuses on Fuzzy Choquet Integral (ChI)-based data fusion methods. Most mathematical approaches for data fusion have focused on combining inputs relative to the assumption of independence between them. However, often there are rich interactions (e.g., correlations) between inputs that should be exploited. The ChI is a powerful aggregation tool that is capable modeling these interactions. Consider the fusion of N sources, where there are 2N unique subsets (interactions); the ChI is capable of learning the worth of each of these possible source subsets. However, the complexity of fuzzy integral-based methods grows quickly, as the fusion of N sources requires training 2N-2 parameters; hence, we require a large amount of training data to avoid the problem of over-fitting. This work addresses the over-fitting problem of ChI-based data fusion with novel regularization strategies. These regularization strategies alleviate the issue of over-fitting while training with limited data and also enable the user to consciously push the learned methods to take a predefined, or perhaps known, structure. Also, the existing methods for training the ChI for decision- and feature-level data fusion involve quadratic programming (QP)-based learning approaches that are exorbitant with their space complexity. This has limited the practical application of ChI-based data fusion methods to six or fewer input sources. This work introduces an online training algorithm for learning ChI. The online method is an iterative gradient descent approach that processes one observation at a time, enabling the applicability of ChI-based data fusion on higher dimensional data sets.
In many real-world data fusion applications, it is imperative to have an explanation or interpretation. This may include providing information on what was learned, what is the worth of individual sources, why a decision was reached, what evidence process(es) were used, and what confidence does the system have on its decision. However, most existing machine learning solutions for data fusion are “black boxes,” e.g., deep learning. In this work, we designed methods and metrics that help with answering these questions of interpretation, and we also developed visualization methods that help users better understand the machine learning solution and its behavior for different instances of data.