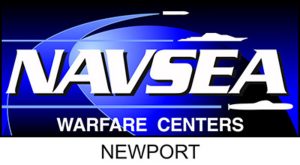
Timothy Havens, the William and Gloria Jackson Associate Professor of Computer Systems, has co-authored a paper recently published in The Journal of the Acoustical Society of America, Volume 50, Issue 1.
The paper is titled, “Recurrent networks for direction-of-arrival identification of an acoustic source in a shallow water channel using a vector sensor.” Havens’s co-authors are Steven Whitaker (EE graduate student), Andrew Barnard (ME-EM/GLRC), and George D, Anderson, US Naval Undersea Warfare Center (NUWC)-Newport.
The work described in the paper was funded by the United States Naval Undersea Warfare Center and Naval Engineering Education Consortium (NEEC) (Grant No. N00174-19-1-0004) and the Office of Naval Research (ONR) (Grant No. N00014-20-1-2793). This is Contribution No. 76 of the Great Lakes Research Center at Michigan Technological University.
Abstract
Conventional direction-of-arrival (DOA) estimation algorithms for shallow water environments usually contain high amounts of error due to the presence of many acoustic reflective surfaces and scattering fields. Utilizing data from a single acoustic vector sensor, the magnitude and DOA of an acoustic signature can be estimated; as such, DOA algorithms are used to reduce the error in these estimations.
Three experiments were conducted using a moving boat as an acoustic target in a waterway in Houghton, Michigan. The shallow and narrow waterway is a complex and non-linear environment for DOA estimation. This paper compares minimizing DOA errors using conventional and machine learning algorithms. The conventional algorithm uses frequency-masking averaging, and the machine learning algorithms incorporate two recurrent neural network architectures, one shallow and one deep network.
Results show that the deep neural network models the shallow water environment better than the shallow neural network, and both networks are superior in performance to the frequency-masking average method.
Citation: The Journal of the Acoustical Society of America 150, 111 (2021); https://doi.org/10.1121/10.0005536Steven Whitaker1,b), Andrew Barnard2, George D. Anderson3, and Timothy C. Havens4
by Allison Mills, University Marketing and Communications
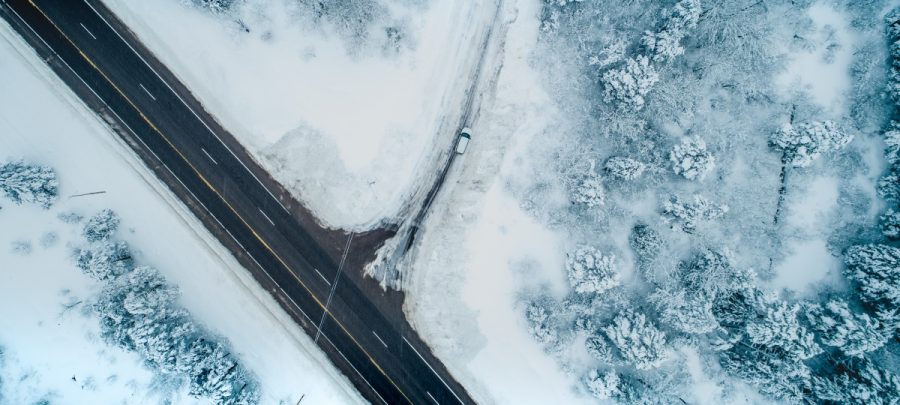
A major challenge for fully autonomous vehicles is navigating bad weather. Snow especially confounds crucial sensor data that helps a vehicle gauge depth, find obstacles and keep on the correct side of the yellow line, assuming it is visible. Averaging more than 200 inches of snow every winter, Michigan’s Keweenaw Peninsula is the perfect place to push autonomous vehicle tech to its limits.
In two papers presented at SPIE Defense + Commercial Sensing 2021, researchers from Michigan Technological University discuss solutions for snowy driving scenarios that could help bring self-driving options to snowy cities like Chicago, Detroit, Minneapolis and Toronto.
The team includes Nathir Rawashdeh and doctoral student Abu-Alrub (CC) as well as Jeremy Bos and student researchers Akhil Kurup, Derek Chopp and Zach Jeffries (ECE).
Read more about their collaborative mobility research on mtu.edu/news.
This MTU news story was published by Science Daily, TechXplore, Knowridge Science Report and other research news aggregators.
Nathir Rawashdeh (AC) led the publication of a paper at the recent online SPIE Defense + Commercial Sensing / Autonomous Systems 2021 Conference.
The paper, entitled “Drivable path detection using CNN sensor fusion for autonomous driving in the snow,” targets the problem of drivable path detection in poor weather conditions including on snow-covered roads. The authors used artificial intelligence to perform camera, radar and LiDAR sensor fusion to detect a drivable path for a passenger car on snow-covered streets. A companion video is available.
Co-authors include Jeremy Bos (ECE).
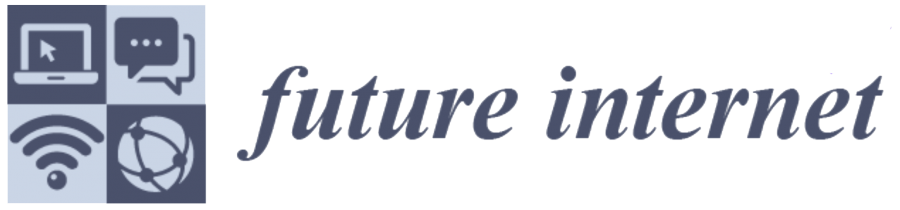
Call for Manuscripts:
Special Issue on Fault Tolerance in Cloud/Edge/Fog Computing in Future Internet, an international peer-reviewed open access monthly journal published by MDPI.
Informational Flyer
https://blogs.mtu.edu/icc/files/2021/04/ali-ebnenasir-call-for-papers-032521-sm.pdf
Deadline
April 20, 2021
Author Notification
June 10, 2021
Website
mdpi.com/journal/futureinternet/special_issues/FT_CEFC
Collection Editors
- Dr. Ali Ebnenasir, Michigan Technological University
- Dr. Sandeep S. Kulkarni, Michigan State University
Keywords
- Fault tolerance
- Cloud computing
- Edge computing
- Resource-constrained devices
- Distributed protocols
- State replication
Topics
Including, but not limited to:
- Faults and failures in cloud and edge computing.
- State replication on edge devices under the scarcity of resources.
- Fault tolerance mechanism on the edge and in the cloud.
- Models for the predication of service latency and costs in distributed fault-tolerant protocols on the edge and in the cloud.
- Fault-tolerant distributed protocols for resource management of edge devices.
- Fault-tolerant edge/cloud computing.
- Fault-tolerant computing on low-end devices.
- Load balancing (on the edge and in the cloud) in the presence of failures.
- Fault-tolerant data intensive applications on the edge and the cloud.
- Metrics and benchmarks for the evaluation of fault tolerance mechanisms in cloud/edge computing.
Background
The Internet of Things (IoT) has brought a new era of computing that permeates in almost every aspect of our lives. Low-end IoT devices (e.g., smart sensors) are almost everywhere, monitoring and controlling the private and public infrastructure (e.g., home appliances, urban transportation, water management system) of our modern life. Low-end IoT devices communicate enormous amount of data to the cloud computing centers through intermediate devices, a.k.a. edge devices, that benefit from stronger computational resources (e.g., memory, processing power).
To enhance the throughput and resiliency of such a three-tier architecture (i.e., low-end devices, edge devices and the cloud), it is desirable to perform some tasks (e.g., storing shared objects) on edge devices instead of delegating everything to the cloud. Moreover, any sort of failure in this three-tier architecture would undermine the quality of service and the reliability of services provided to the end users.
Scope
Theoretical and experimental methods that incorporate fault tolerance in cloud and edge computing, which have the potential to improve the overall robustness of services in three-tier architectures.
Manuscript Submission Information
Manuscripts should be submitted online at www.mdpi.com by registering and logging in to this website (https://www.mdpi.com/user/login/). Once you are registered, click here to go to the submission form (https://susy.mdpi.com/user/manuscripts/upload/?journal=futureinternet).
Manuscripts can be submitted until the deadline. All papers will be peer-reviewed. Accepted papers will be published continuously in the journal (as soon as accepted) and will be listed together on the special issue website. Research articles, review articles as well as short communications are invited. For planned papers, a title and short abstract (about 100 words) can be sent to the Editorial Office for announcement on this website.
Submitted manuscripts should not have been published previously, nor be under consideration for publication elsewhere (except conference proceedings papers). All manuscripts are thoroughly refereed through a single-blind peer-review process. A guide for authors and other relevant information for submission of manuscripts is available on the Instructions for Authors page.
Please visit the Instructions for Authors page before submitting a manuscript.
The Article Processing Charge (APC) for publication in this open access journal is 1400 CHF (Swiss Francs). Submitted papers should be well formatted and use good English.
Authors may use MDPI’s English editing service prior to publication or during author revisions.