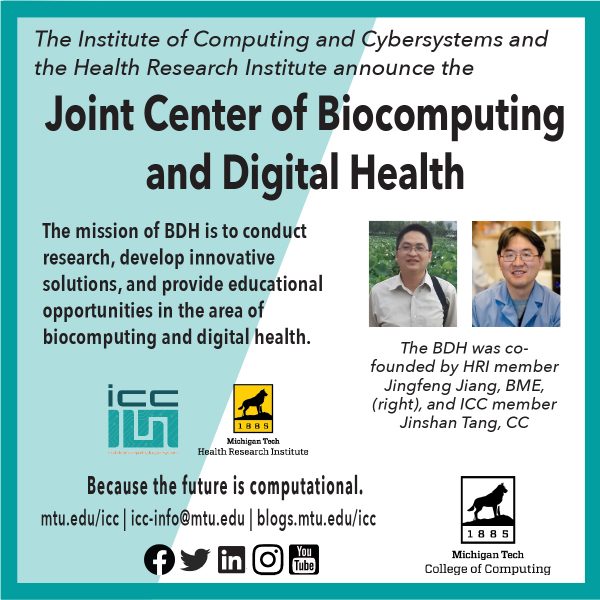
The Institute of Computing and Cybersystems (ICC) and the Health Research Institute (HRI) have established the Joint Center of Biocomputing and Digital Health (BDH).
The new research institute was co-founded by HRI member Jingfeng Jiang (BME) and ICC member Jinshan Tang (CC).
The mission of Joint Center of Biocomputing and Digital Health (BDH) is to conduct research, develop innovative solutions, and provide educational opportunities in the area of biocomputing and digital health, thereby enhancing Michigan Tech’s ability to recruit and retain high-quality researchers and students, elevating Michigan Tech’s presence in developing technologies for healthcare delivery, and increasing knowledge sharing in the global community.
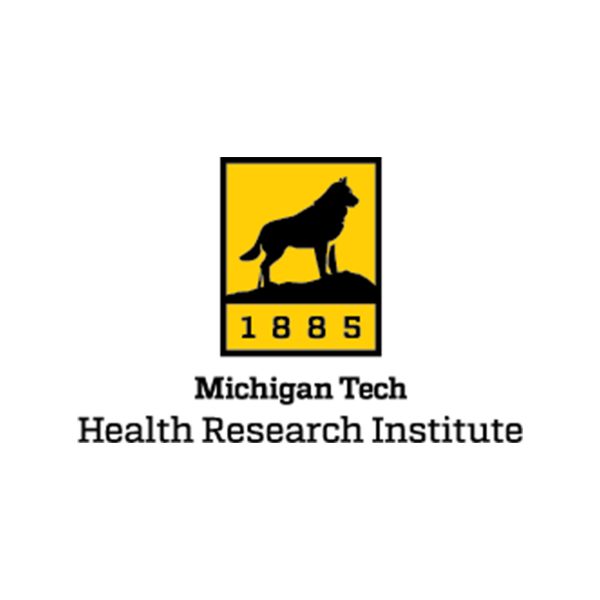
Jingfeng Jiang is a professor with the Department of Biomedical Engineering. His research interests are in biomechanics, automated control of ultrasound scanning including the use of 3D printing technology, image and signal processing, non-invasive assessment of biomechanical properties of soft tissues, and computer aided analyses of cardiovascular flow.
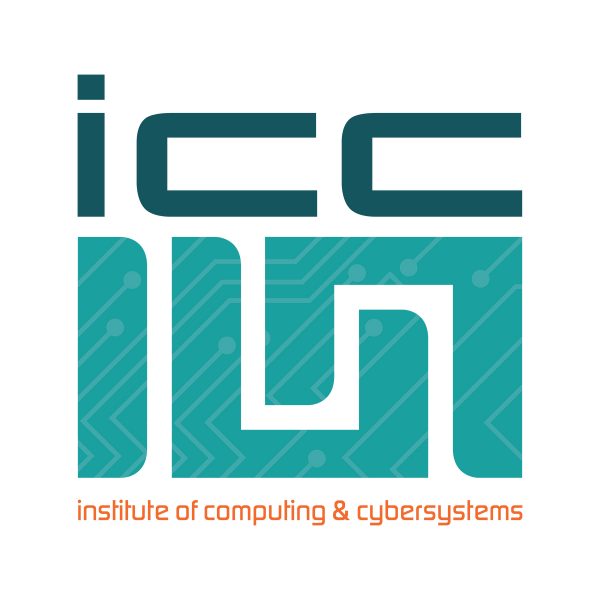
Jinshan Tang is a professor in the Department of Applied Computing. His research interests are in image processing and pattern recognition, biomedical imaging and medical image analysis, and medical informatics and intelligent medical diagnosis systems. Tang is a member of the ICC’s Center for Cyber-Physical Systems.
The Institute of Computing and Cybersystems (ICC) creates and supports an arena in which faculty and students work collaboratively across organizational boundaries in an environment that mirrors contemporary technological innovation.
The Health Research Institute (HRI) aims to establish and maintain a thriving environment that promotes translational, interdisciplinary, and increasingly convergent health-related research and inspires education and outreach activities.
Please contact Jingfeng Jiang (jjiang1@mtu.edu) with questions.