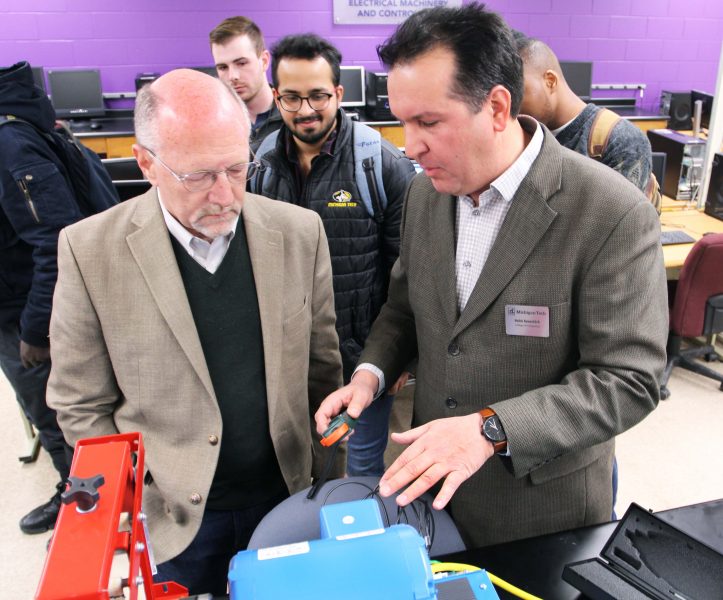
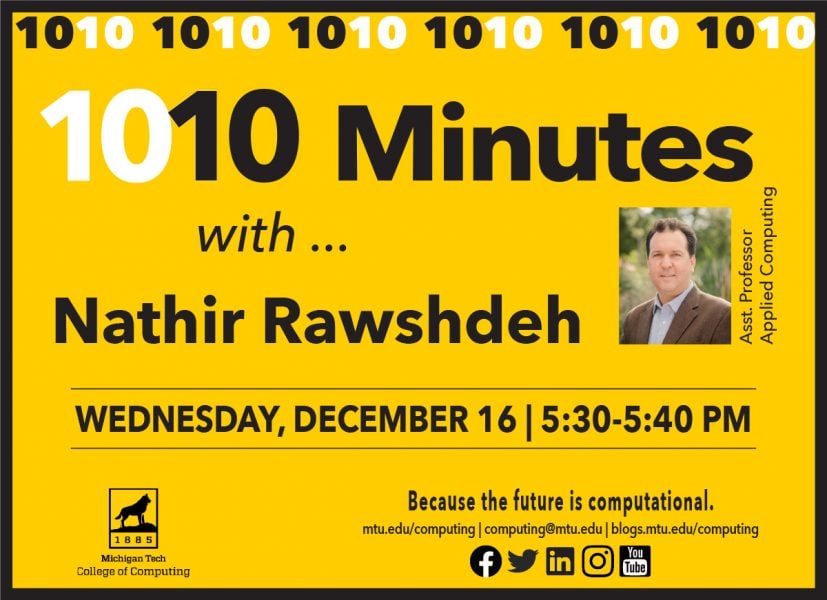
You are invited to spend one-zero-one-zero—that is, ten—minutes with Dr. Nathir Rawashdeh on Wednesday, December 16, from 5:30 to 5:40 p.m.
Rawashdeh is assistant professor of applied computing in the College of Computing at Michigan Tech.
He will present his current research work, including the using artificial intelligence for autonomous driving on snow covered roads, and a mobile robot using ultraviolet light to disinfect indoor spaces. Following, Rawashdeh will field listener questions.
We look forward to spending 1010 minutes with you!