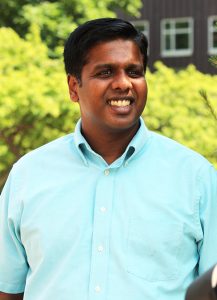
Thomas Oommen (DataS), associate professor of geological and mining engineering and sciences, was recently featured in a Michigan Tech Unscripted Research Blog titled, ” Geohazards on the Horizon.”
Oommen was part of a US team in Mumbai this August working on disaster management curriculum with the Tata Institute of Social Sciences (TISS), the only institution in all of Mumbai—one of the world’s largest cities with 19 million people—to offer a degree in disaster management.
Oommen’s trip was funded by the US Consulate General in Mumbai. Read more about the team’s work on the Unscripted blog here: https://www.mtu.edu/unscripted/stories/2019/august/geohazards-on-the-horizon.html