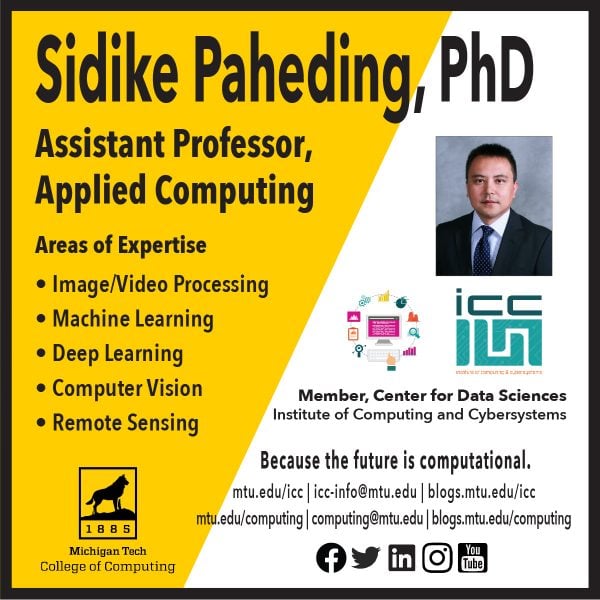
A paper co-authored by Sidike Paheding, Applied Computing, has been published in the journal, IEEE Access. “Trends in Deep Learning for Medical Hyperspectral Image Analysis,” was available for early access on March 24, 2021.
The paper discusses the implementation of deep learning for medical hyperspectral imaging.
Co-authors of the paper are Uzair Khan, Colin Elkin, and Vijay Devabhaktuni, all with the Department of Electrical and Computer Engineering, Purdue University Northwest.
Abstract
Deep learning algorithms have seen acute growth of interest in their applications throughout several fields of interest in the last decade, with medical hyperspectral imaging being a particularly promising domain. So far, to the best of our knowledge, there is no review paper that discusses the implementation of deep learning for medical hyperspectral imaging, which is what this work aims to accomplish by examining publications that currently utilize deep learning to perform effective analysis of medical hyperspectral imagery.
This paper discusses deep learning concepts that are relevant and applicable to medical hyperspectral imaging analysis, several of which have been implemented since the boom in deep learning. This will comprise of reviewing the use of deep learning for classification, segmentation, and detection in order to investigate the analysis of medical hyperspectral imaging. Lastly, we discuss the current and future challenges pertaining to this discipline and the possible efforts to overcome such trials.
DOI: 10.1109/ACCESS.2021.3068392
IEEE Access is a multidisciplinary, applications-oriented, all-electronic archival journal that continuously presents the results of original research or development across all of IEEE’s fields of interest. Supported by article processing charges, its hallmarks are a rapid peer review and publication process with open access to all readers.