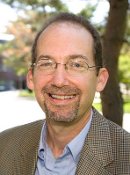
University Professor Timothy Schulz (ECE) will be featured at the Michigan Tech Research Forum (MTRF) at 4:30 p.m. Wednesday, Oct. 14.
Schulz’s presentation is titled “Direct Measurement of Coherent Fields.” Additional details can be found on the MTRF website.
The presentation will be available via Zoom and a limited number of people will be permitted to attend in person, dependent on university guidelines on the date of the event. If you wish to be considered for in-person attendance, complete this form by today (Oct. 9).
Schulz is a member of the ICC’s Center for Data Sciences.
The MTRF is presented by the Office of the Provost in coordination with the Office of the Vice President for Research. The forum showcases and celebrates the work of Michigan Tech researchers and aims to strengthen discussions in our community. All are welcome, including the general public.