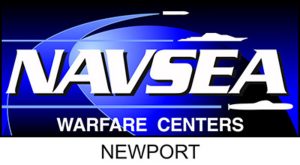
Timothy Havens, the William and Gloria Jackson Associate Professor of Computer Systems, has co-authored a paper recently published in The Journal of the Acoustical Society of America, Volume 50, Issue 1.
The paper is titled, “Recurrent networks for direction-of-arrival identification of an acoustic source in a shallow water channel using a vector sensor.” Havens’s co-authors are Steven Whitaker (EE graduate student), Andrew Barnard (ME-EM/GLRC), and George D, Anderson, US Naval Undersea Warfare Center (NUWC)-Newport.
The work described in the paper was funded by the United States Naval Undersea Warfare Center and Naval Engineering Education Consortium (NEEC) (Grant No. N00174-19-1-0004) and the Office of Naval Research (ONR) (Grant No. N00014-20-1-2793). This is Contribution No. 76 of the Great Lakes Research Center at Michigan Technological University.
Abstract
Conventional direction-of-arrival (DOA) estimation algorithms for shallow water environments usually contain high amounts of error due to the presence of many acoustic reflective surfaces and scattering fields. Utilizing data from a single acoustic vector sensor, the magnitude and DOA of an acoustic signature can be estimated; as such, DOA algorithms are used to reduce the error in these estimations.
Three experiments were conducted using a moving boat as an acoustic target in a waterway in Houghton, Michigan. The shallow and narrow waterway is a complex and non-linear environment for DOA estimation. This paper compares minimizing DOA errors using conventional and machine learning algorithms. The conventional algorithm uses frequency-masking averaging, and the machine learning algorithms incorporate two recurrent neural network architectures, one shallow and one deep network.
Results show that the deep neural network models the shallow water environment better than the shallow neural network, and both networks are superior in performance to the frequency-masking average method.
Citation: The Journal of the Acoustical Society of America 150, 111 (2021); https://doi.org/10.1121/10.0005536Steven Whitaker1,b), Andrew Barnard2, George D. Anderson3, and Timothy C. Havens4
by Pasi Lautala
Thomas Oommen (GMES, ICC), Ricardo Eiris, (CEGE, ICC), and Beth Veinott (CLS, ICC) are among eight Michigan Tech researchers who have submitted a a record number of eight concept papers for proposed research projects with the Federal Railroad Administration.
The Federal Railroad Administration (FRA) requested that Michigan Tech submit a record number of eight concept papers for proposed research projects as part of their 2021 Broad Agency Announcement.
In addition, Tech is a subcontractor for two more concept paper proposals. The paper submittal was coordinated by the Rail Transportation Program and the range of topics speaks to the diversity of Michigan Tech’s expertise applicable to the rail transportation. The PIs are looking forward to FRA decisions on how many of these papers advance to full proposals.
Each of the 10 projects had a different principal investigator (PI), representing six university departments/institutes and several more co-PIs.
The project titles and their PIs include:
- Hyper- and Multi-spectral Sensing and Deep Learning for Automated Identification of Roadbed Condition, (PI, Thomas Oommen, GMES).
- Wire Arc Additive Manufacturing (WAAM) for Weld Enhanced Cast Steel Coupler Knuckles (PI, Paul Sanders, MSE).
- IoT Assisted Data-analytics Framework Enables Assessment of Location Based Ride Quality (LBRQ) (PI, Sriram Malladi, MEEM).
- RailStory: Using Web-based Immersive Storytelling to Attract the Next Generation of Young Women in Rail (PI, Ricardo Eiris, CEGE).
- A Risk Informed Decision-Making Framework for Coastal Railroad System Subjected to Storm Hazards and Sea Level Rise (PI, Yousef Darestani, CEGE).
- Rail Corridor Life-Cycle Assessment (LCA) Framework, Factors and Models to Support Project Evaluation and Multi-Modal Comparisons (PI, Pasi Lautala, CEGE).
- Development of Infrared Thermography for Effective Rail Weld Inspection (PI, Qingli Dai, CEGE).
- Enabling Longer-distance, AI-enabled Drone-based Grade Crossing Assessment in Potentially GPS Denied Environments (PI, Colin Brooks).
- Multi-Site Simulation to Examine Driver Behavior Impact of Integrated Rail Crossing Violation Warning (RCVW) and In-Vehicle Auditory/Visual Alert (IVAA) System (PI, Elizabeth Veinott, subcontract with Virginia Tech).
- Evaluation of Non-traditional Methods of Reducing Emissions in Short Line Railroad Operations (PI, Jeremy Worm, subcontract with ASLRRA).
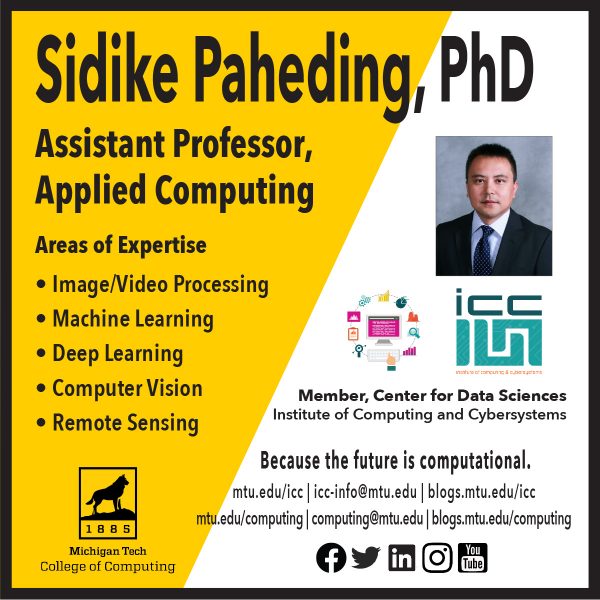
Dr. Sidike Paheding, assistant professor of Applied Computing, is the co-author of a paper published June 3, 2021, the journal “IEEE Access.” The paper is titled, “U-net and its variants for medical image segmentation: A review of theory and applications.”
The paper discusses U-net, an image segmentation technique developed primarily for image segmentation tasks.
The co-authors of the paper are Nahian Siddique, Colin P. Elkin, and Vijay Devabhaktuni, all with the Department of Electrical and Computer Engineering, Purdue University Northwest, Hammond, Indiana.
Abstract
U-net is an image segmentation technique developed primarily for image segmentation tasks. These traits provide U-net with a high utility within the medical imaging community and have resulted in extensive adoption of U-net as the primary tool for segmentation tasks in medical imaging. The success of U-net is evident in its widespread use in nearly all major image modalities, from CT scans and MRI to Xrays and microscopy. Furthermore, while U-net is largely a segmentation tool, there have been instances of the use of U-net in other applications. Given that U-net’s potential is still increasing, this narrative literature review examines the numerous developments and breakthroughs in the U-net architecture and provides observations on recent trends. We also discuss the many innovations that have advanced in deep learning and discuss how these tools facilitate U-net. In addition, we review the different image modalities and application areas that have been enhanced by U-net.
The paper can be accessed on the IEEE Access website.
IEEE Access is a multidisciplinary, applications-oriented, all-electronic archival journal that continuously presents the results of original research or development across all of IEEE’s fields of interest. Supported by article processing charges, its hallmarks are a rapid peer review and publication process with open access to all readers.
by Allison Mills, University Marketing and Communications
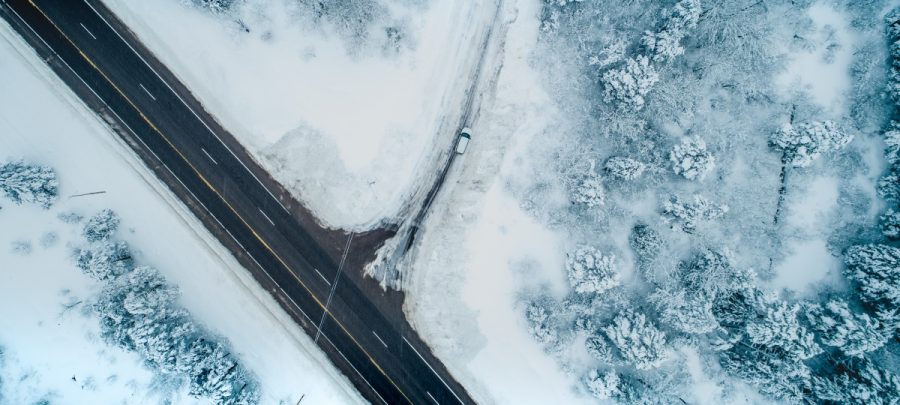
A major challenge for fully autonomous vehicles is navigating bad weather. Snow especially confounds crucial sensor data that helps a vehicle gauge depth, find obstacles and keep on the correct side of the yellow line, assuming it is visible. Averaging more than 200 inches of snow every winter, Michigan’s Keweenaw Peninsula is the perfect place to push autonomous vehicle tech to its limits.
In two papers presented at SPIE Defense + Commercial Sensing 2021, researchers from Michigan Technological University discuss solutions for snowy driving scenarios that could help bring self-driving options to snowy cities like Chicago, Detroit, Minneapolis and Toronto.
The team includes Nathir Rawashdeh and doctoral student Abu-Alrub (CC) as well as Jeremy Bos and student researchers Akhil Kurup, Derek Chopp and Zach Jeffries (ECE).
Read more about their collaborative mobility research on mtu.edu/news.
This MTU news story was published by Science Daily, TechXplore, Knowridge Science Report and other research news aggregators.
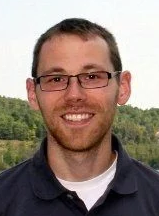
The Institute of Computing and Cybersystems (ICC) is pleased to welcome Tony Pinar as a member. Pinar’s primary research interests are in applied machine learning and data fusion.
A lecturer in Michigan Tech’s Electrical and Computer Engineering department, Pinar holds a Ph.D. and M.S. in Electrical Engineering from Michigan Tech. His previous positions include research engineer for Michigan Tech’s Advanced Power System Research Center and electrical design engineer for GE Aviation. He is a member of the Institute of Electrical and Electronics Engineers (IEEE) and the IEEE Computational Intelligence Society.
Pinar’s teaching interests include machine learning, signal processing, and electronic design. Included among the classes he teaches are Electronics, Electronic Applications, Probability—Signal Analysis, and Control Systems I.
“Teaching is like a puzzle where one may have to take a difficult concept, reduce it to digestible pieces, and deliver them to fresh minds in a way to maximize understanding and insight,” Pinar says. “That challenge is what drives me to be a better teacher.”
Pinar believes that to be a good teacher one must understand the topics very well and he strives for the most effective delivery. “This keeps me on my toes, forces me to constantly identify holes in my knowledge, and drives me to continuously strive to learn new things,” he explains.
On research, Pinar says it is rewarding to work on open-ended and novel problems that are in their infancy and at the cutting edge of today’s technology.
“It is also exciting to me to watch the cutting edge move forward, see what sticks and what doesn’t, and observe how the direction(s) of the field evolve,” he adds. “I’m very new to this domain so I haven’t been able to observe it for long, but I am looking forward to witnessing the future of the field.”
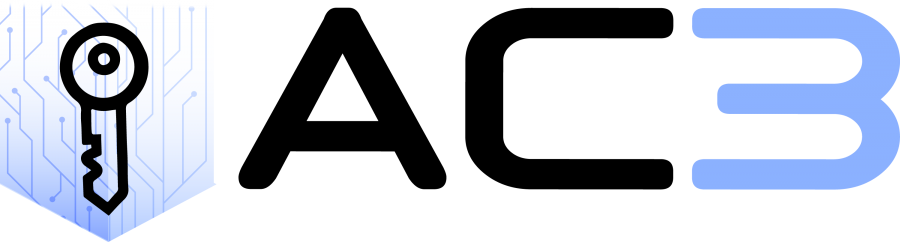
The 2021 EAI International Conference on Applied Cryptography in Computer and Communications (AC3 2021) takes place May 15-16, 2021.
Dr. Bo Chen, Computer Science, founding general chair of the new EAI conference, says the conference has brought together researchers, developers and practitioners from around the world who will focus on, discuss, and explore the area of applied cryptography in computer and communication systems.
Conference Topics
Conference topics include all aspects of applied cryptography, including symmetric cryptography, public-key cryptography, cryptographic protocols, cryptographic implementations, cryptographic standards and practices, as well as using cryptography to solve real-world problems.
Technical Program
The AC3 2021 technical program includes four main conference tracks at which 11 papers will be presented virtually in oral presentations.
- Track 1 – Blockchain
- Track 2 – Authentication
- Track 3 – Secure Computation
- 4 – Practical Crypto Application. Aside from the high-quality technical paper presentations, the technical program also features two keynote speeches, and one technical workshop.
Keynotes
The two keynote speeches will be delivered by Prof. Kui Ren (ACM Fellow, IEEE Fellow), Zhejiang University, China; and IEEE Fellow Prof. Robert Deng, Singapore Management University.
Workshop
A workshop, the First International Workshop on Security for Internet of Things (IOTS 2021), includes four technical papers which aim to develop cryptographic techniques for ensuring the IoT security. The conference, originally planned to be held in Xiamen China, was moved it online for the health and safety of participants.
Register to participate in the virtual conference here. Use the “Sign up for free access to the livestream” option.
European Alliance for Innovation (EAI) is an international professional community and a nonprofit organization. The goal of EAI is to empower the global ICT research and innovation community, and to promote cooperation between European and International ICT communities.
EAI Conferences span the globe with opportunities to meet, explore, and contribute to the world of ICT research. With 100+ annual events (including MobiQuitous, SecureComm, etc.), EAI is one of the world’s most prolific scientific communities.
EAI Conferences are published via Springer’s LNICST and EAI’s EUDL, and they are indexed in all leading indexing services, including EI, ISI, Scopus, CrossRef, Google Scholar, dblp, MAS, EBSCO, Microsoft Academic Search, CiteSeerX, and more.
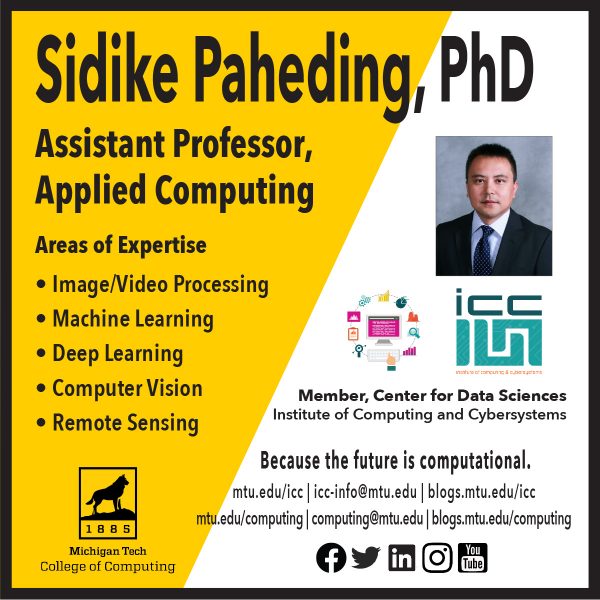
Sidike Paheding (AC/ICC) is the principal investigator on a project that has received a $19,037 research and development grant from Purdue University. The two-year project is titled, “Cybersecurity Modules Aligned with Undergraduate Computer Science and Engineering Curricula.”
The project aims to serve the national interest by improving how cybersecurity concepts are taught in undergraduate computing curricula.
The grant is a sub-award of a $159,417 Purdue University NSF project . View that project here.
Abstract
This project aims to serve the national interest by improving how cybersecurity concepts are taught in undergraduate computing curricula. The need to design and maintain cyber-secure computing systems is increasingly important. As a result, the future technology workforce must be trained to have a security mindset, so that they consider cybersecurity during rather than after system design. This project aims to achieve this goal by building plug-and-play, hands-on cybersecurity modules for core courses in Computer Engineering, and Computer Science and Engineering. The modules will align with the curricula recommended by the Association for Computing Machinery and will be designed for easy adoption into computing programs nationwide. Modules will be designed for integration into both introductory and advanced courses, thus helping students develop in-depth understanding of cybersecurity as they progress through their computing curriculum. It is expected that the project will encourage more students to pursue careers or higher degrees in the field of cybersecurity.
The project will examine how the modules may be best integrated into existing curricula and the effects of the modules on student learning and interest in cybersecurity. Assessment will leverage several methods including (a) a task load index to quantify rigor, (b) surveys to gain insight into the development of students’ security mindset and perceptions of cybersecurity, and (c) analysis of learning using analytical course rubrics. Deliverables of this project will include a suite of plug-and-play cybersecurity modules for Computer Engineering and Computer Science and Engineering courses that span from introductory to advanced levels and that meet standards for content breadth and depth. The results will be disseminated through publications, presentations, press releases, and social media to ensure that project outcomes are shared widely. The NSF Improving Undergraduate STEM Education: Education and Human Resources Program supports research and development projects to improve the effectiveness of STEM education for all students. Through the Engaged Student Learning track, the program supports the creation, exploration, and implementation of promising practices and tools.
Nathir Rawashdeh (AC) led the publication of a paper at the recent online SPIE Defense + Commercial Sensing / Autonomous Systems 2021 Conference.
The paper, entitled “Drivable path detection using CNN sensor fusion for autonomous driving in the snow,” targets the problem of drivable path detection in poor weather conditions including on snow-covered roads. The authors used artificial intelligence to perform camera, radar and LiDAR sensor fusion to detect a drivable path for a passenger car on snow-covered streets. A companion video is available.
Co-authors include Jeremy Bos (ECE).